Confidence that quantum computers will solve major problems beyond the reach of traditional computers—a milestone known as quantum advantage—has soared in the past twelve months. Equity investments in quantum computing nearly tripled in 2020, the busiest year on record, and are set to rise even further in 2021. (See Exhibit 1.) In 2021, IonQ became the first publicly traded pure-play quantum computing company, at an estimated initial valuation of $2 billion.
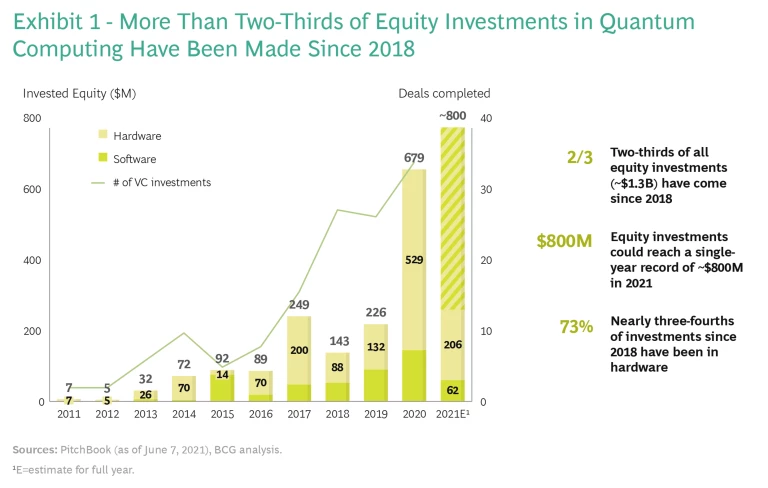
It’s not just financial investors. Governments and research centers are ramping up investment as well. Cleveland Clinic, University of Illinois Urbana-Champaign and the Hartree Centre have each entered into “discovery acceleration” partnerships with IBM—anchored by quantum computing—that have attracted $1 billion in investment. The $250 billion U.S. Innovation and Competition Act, which enjoys broad bipartisan support in both houses of the US Congress, designates quantum information science and technology as one of ten key focus areas for the National Science Foundation.
Potential corporate users are also gearing up. While only 1% of companies actively budgeted for quantum computing in 2018, 20% are expected to do so by 2023, according to Gartner.
Three factors are driving the rising interest. The first is technical achievement. Since we released our last report on the market outlook for quantum computing in May 2019, there have been two highly publicized demonstrations of “quantum supremacy”—one by Google in October 2019 and another by a group at the University of Science and Technology of China in
In the past two years, nearly every major quantum computing technology provider has released a roadmap setting out the critical milestones along the path to quantum advantage over the next decade.
BCG has been tracking developments in the technology and business of quantum computing for several years. (See the box, BCG on Quantum Computing.) This report takes a current look at the evolving market, especially with respect to the timeline to quantum advantage and the specific use cases where quantum computing will create the most value. We have updated our 2019 projections and looked into the economics of more than 20 likely use cases. We have added detail to our technology development timeline, informed by what the technology providers themselves are saying about their roadmaps, and we have refreshed our side-by-side comparison of the leading hardware technologies. We also offer action plans for financial investors, corporate and government end users, and tech providers with an interest in quantum computing. They all need to understand a complex and rapidly evolving landscape as they plan when and where to place their bets.
BCG on Quantum Computing
The Coming Quantum Leap in Computing (May 2018)
The Next Decade in Quantum Computing—and How to Play (November 2018)
Where Will Quantum Computing Create Value—and When? (May 2019)
Will Quantum Computing Transform Biopharma R&D? (December 2019)
A Quantum Advantage in Fighting Climate Change (January 2020)
It’s Time for Financial Institutions to Place Their Quantum Bets (October 2020)
TED Talk: The Promise of Quantum Computers (February 2021)
Ensuring Online Security in a Quantum Future (March 2021)
Applications and Use Cases
Quantum computers will not replace the traditional computers we all use now. Instead they will work hand-in-hand to solve computationally complex problems that classical computers can’t handle quickly enough by themselves. There are four principal computational problems for which hybrid machines will be able to accelerate solutions—building on essentially one truly “quantum advantaged” mathematical function. But these four problems lead to hundreds of business use cases that promise to unlock enormous value for end users in coming decades.
BCG estimates that quantum computing could create value of $450 billion to $850 billion in the next 15 to 30 years. Value of $5 billion to $10 billion could start accruing to users and providers as soon as the next three to five years if the technology scales as fast as promised by key vendors.
BCG estimates that quantum computing could create value of $450 billion to $850 billion in the next 15 to 30 years. Value of $5 billion to $10 billion could start accruing to users and providers as soon as the next three to five years.
There is no consensus on the exhaustive set of problems that quantum computers will be able to tackle, but research is concentrated on the following types of computational problems:
- Simulation: Simulating processes that occur in nature and are difficult or impossible to characterize and understand with classical computers today. This has major potential in drug discovery, battery design, fluid dynamics, and derivative and option pricing.
- Optimization: Using quantum algorithms to identify the best solution among a set of feasible options. This could apply to route logistics and portfolio risk management.
- Machine learning (ML): Identifying patterns in data to train ML algorithms. This could accelerate the development of artificial intelligence (for autonomous vehicles, for example) and the prevention of fraud and money-laundering.
- Cryptography: Breaking traditional encryption and enabling stronger encryption standards, as we detailed in a recent report.
These computational problems could unlock use cases in multiple industries, from finance to pharmaceuticals and automotive to aerospace. (See Exhibit 2.) Consider the potential in pharmaceutical R&D. The average cost to develop a new drug is about $2.4 billion. Pre-clinical research selects only about 0.1% of small molecules for clinical trials, and only about 10% of clinical trials result in a successful product. A big barrier to improving R&D efficiency is that molecules undergo quantum phenomena that cannot be modeled by classical computers.
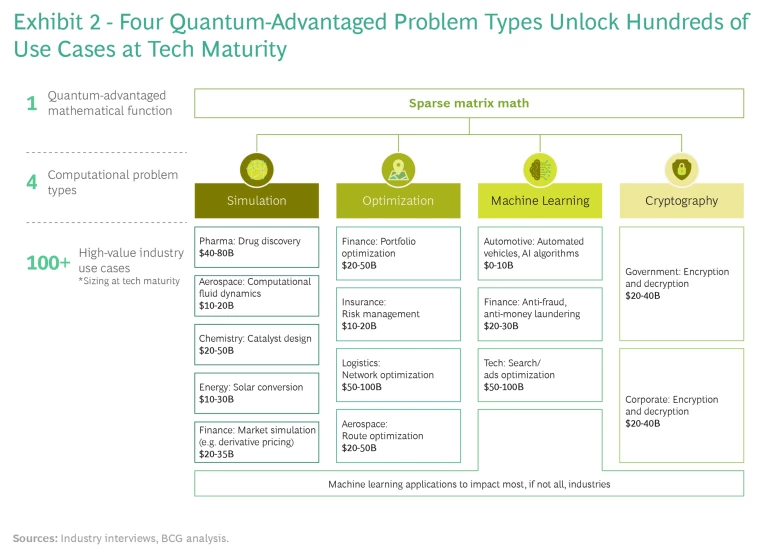
Quantum computers, on the other hand, can efficiently model a practically complete set of possible molecular interactions. This is promising not only for candidate selection, but also for identifying potential adverse effects via modeling (as opposed to having to wait for clinical trials) and even, in the long term, for creating personalized oncology drugs. For a top pharma company with an R&D budget in the $10 billion range, quantum computing could represent an efficiency increase of up to 30%. Assuming the company captures 80% of this value (with the balance going to its quantum technology partners), this means savings on the order of $2.5 billion and increase in operating profit of up to 5%.
Or think about prospects for financial institutions. Every year, according to the Bank for International Settlements, more than $10 trillion worth of options and derivatives are exchanged globally. Many are priced using Monte Carlo techniques—calculating complex functions with random samples according to a probability distribution. Not only is this approach inefficient, it also lacks accuracy, especially in the face of high tail risk. And once options and derivatives become bank assets, the need for high-efficiency simulation only grows as the portfolio needs to be re-evaluated continuously to track the institution’s liquidity position and fresh risks. Today this is a time-consuming exercise that often takes 12 hours to run, sometimes much more. According to a former quantitative trader at BlackRock, “Brute force Monte Carlo simulations for economic spikes and disasters can take a whole month to run.” Quantum computers are well-suited to model outcomes much more efficiently. This has led Goldman Sachs to team up with QC Ware and IBM with a goal of replacing current Monte Carlo capabilities with quantum algorithms by 2030.
For a top pharma company with an R&D budget in the $10 billion range, quantum computing could represent an efficiency increase of up to 30%.
Exhibit 3 shows our estimates for the projected value of quantum computing in each of the four major problem types and the range of value in more than 20 priority use cases once the technology is mature.
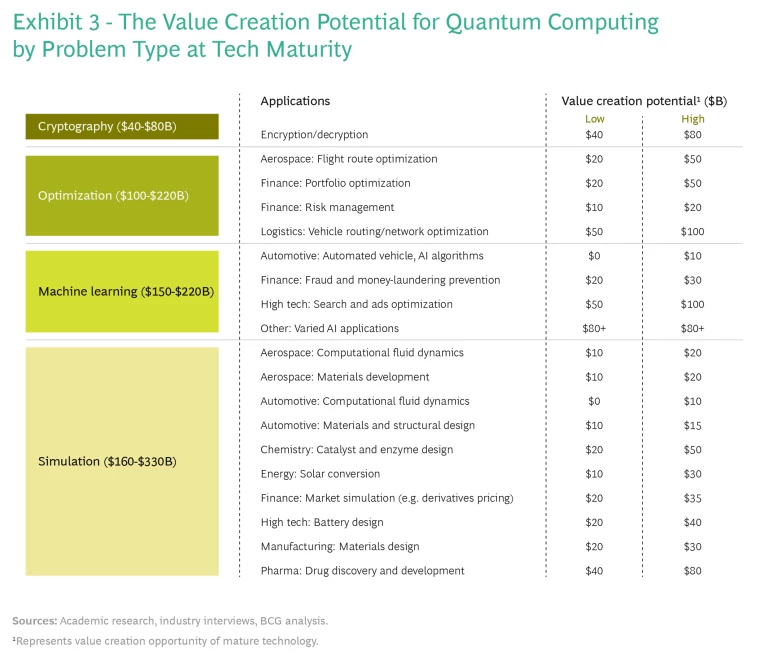
It should be noted that quantum computers do have limitations, some of which are endemic to the technology. They are disadvantaged, for example, relative to classical computers on many fundamental computation types such as arithmetic. As a result they are likely to be best used in conjunction with classical computers in a hybrid configuration rather than on a standalone basis, and they will be used to perform calculations (such as optimizing) rather than execute commands (such as streaming a movie). Moreover, creating a quantum state from classical data currently requires a high number of operations, potentially limiting big data use cases (unless hybrid encoding solutions or a form of quantum RAM can be developed).
Other limitations may be overcome in time. Foremost among these is fabricating the quantum bits, or qubits, that power the computers. This is difficult in part because qubits are highly noisy and sensitive to their environment. Superconducting qubits, for example, require temperatures near absolute zero. Qubits are also highly unreliable. Thousands of error-correcting qubits can be required for each qubit used for calculation. Many companies, such as Xanadu, IonQ, and IBM, are targeting a million-qubit machine as early as 2025 in order to unlock 100 qubits available for calculation, using a common 10,000:1 “overhead” ratio as a rule of thumb.
Rising concerns about computing’s energy consumption and its effects on climate change raise questions about quantum computers’ future impact.
Rising concerns about computing’s energy consumption and its effects on climate change raise questions about quantum computers’ future impact. So far, it is extremely small relative to classical computers because quantum computers are designed to minimize qubit interactions with the environment. Control equipment outside the computer (such as the extreme cooling of superconducting computers) typically requires more power than the machine itself. Sycamore, Google’s 53-qubit computer that demonstrated quantum supremacy, consumes a few kWh of electricity in a short time (200 seconds), compared with supercomputers that typically require many MWh of electric power.
Three Stages of Development
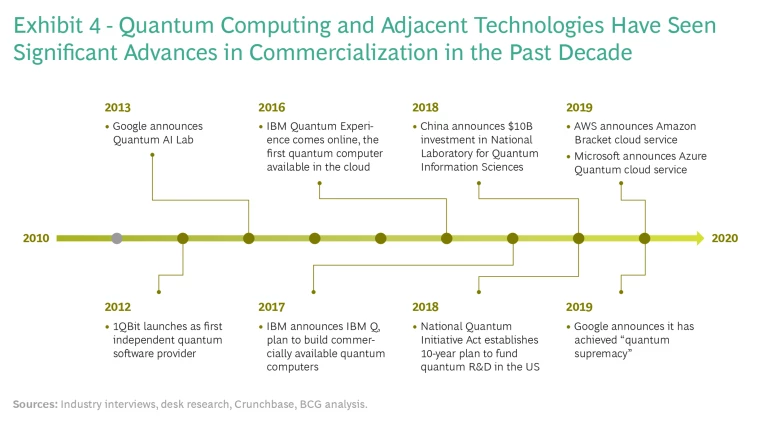
Quantum computing has made long strides in the last decade, but it is still in the early stages of development, and broad commercial application is still years away. (See Exhibit 4.) We are currently in the stage commonly known as the “NISQ” era, for Noisy Intermediate Scale Quantum technology. Systems of qubits have yet to be “error-corrected,” meaning that they still lose information quickly when exposed to noise. This stage is expected to last for the next three to ten years. Even during this early and imperfect time, researchers hope that a number of use cases will start to mature. These include efficiency gains in the design of new chemicals, investment portfolio optimization, and drug discovery.
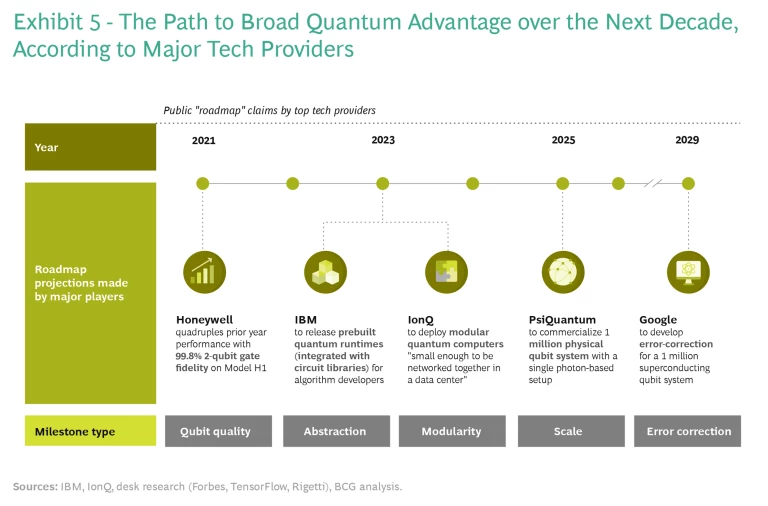
The NISQ era is expected to be followed by a five- to 20-year period of broad quantum advantage once the error correction issues have been largely resolved. The path to broad quantum advantage has become clearer as many of the major players have recently released quantum computing roadmaps. (See Exhibit 5.) Besides error correction, the milestones to watch for over the next decade include higher quality qubits, the development of abstraction layers for model developers, at-scale and modular systems, and the scaling up of machines. But even if these ambitious targets are reached, further progress is necessary to achieve quantum advantage. (Some believe that qubit fidelity, for example, will have to improve by several orders of magnitude beyond even the industry-leading ion traps in Honeywell’s Model H1.) It is unlikely that any single player will put it all together in the next five years. Still, once developers reach the five key milestones, which taken together make up the essential ingredients of broad quantum advantage, the race will be on to modularize and scale the most advanced architectures to achieve full-scale fault tolerance in the ensuing decades.
While new use cases are expected to become available as the technology matures, they are unlikely to emerge in a steady or linear manner. A scale breakthrough, such as the million-qubit machine that PsiQuantum projects to release in 2025, would herald a step change in capability. Indeed, early technology milestones will have a disproportionate impact on the overall timeline, as they can be expected to safeguard against a potential “quantum winter” scenario, in which investment dries up, as it did for AI in the late 1980s. Because its success is so closely aligned to ongoing fundamental science, quantum computing is a perfect candidate for a timeline-accelerating discovery that could come at any point.
Because its success is so closely aligned to ongoing fundamental science, quantum computing is a perfect candidate for a timeline-accelerating discovery that could come at any point.
Five Hardware Technologies
One of the big questions surrounding quantum computing is which hardware technology will win the race. At the moment five well-funded and well-researched candidates are in the running: superconductors, ion traps, photonics, quantum dots and cold atoms. All of these were developed in groundbreaking physical experiments and realizations of the 1990s.
Superconductors and ion traps have received the most attention over the past decade. Technology leaders such as IBM, Google, and recently Amazon Web Services are developing superconducting systems that are based on superpositions of currents simultaneously flowing in opposite directions around a superconductor. These systems have the benefit of being relatively easy to manufacture (they are solid state), but they have short coherence times and require extremely low temperatures.
Honeywell and IonQ are leading the way on trapped ions, so named because the qubits in this system are housed in arrays of ions that are trapped in electric fields while their quantum states are controlled by lasers. Ion traps are less prone to defects than superconductors, leading to higher qubit lifetimes and gate fidelities, but accelerating gate operation time and scaling beyond a single trap are key challenges yet to be overcome.
Photonics have risen in prominence recently, partly because of their compatibility with silicon chip-making capabilities (in which the semiconductor industry has invested $1 trillion for R&D over the past 50 years) and widely available telecom fiber optics. Photonics companies, such as Xanadu and PsiQuantum (currently the most well-funded private quantum computing company, with $275 million), are developing systems in which qubits are encoded in the quantum states of photons moving along circuits in silicon chips and networked by fiber optics. Photonic qubits are resistant to interference and will thus be much easier to error-correct. Overcoming photon losses due to scattering remains a key challenge.
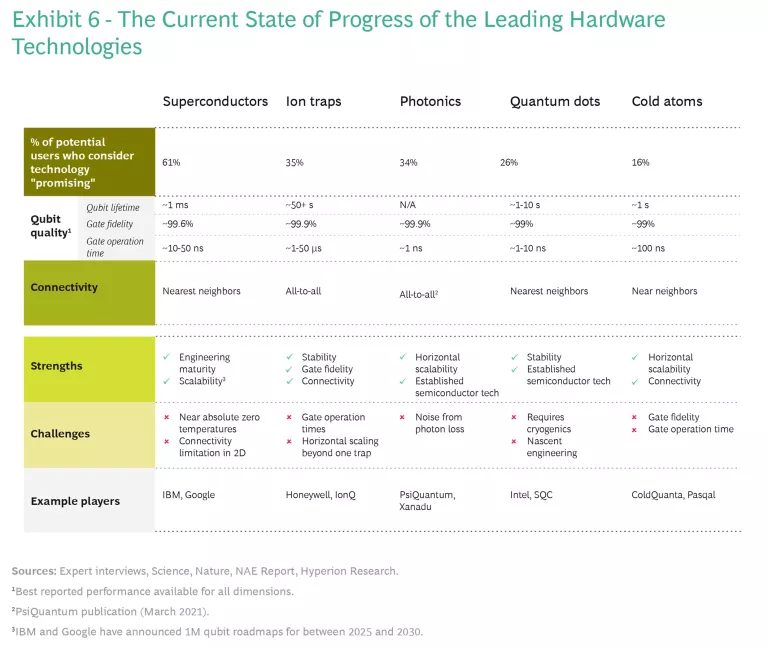
Companies leading research into quantum dots, such as Intel and SQC, are developing systems in which qubits are made from spins of electrons or nuclei fixed in a solid substrate. Benefits include long qubit lifetimes and leveraging silicon chip-making, while the principal drawback is a proneness to interference that currently results in low gate fidelities.
Cold atoms leverage a technique similar to ion traps, except that qubits are made from arrays of neutral atoms—rather than ions—trapped by light and controlled by lasers. Notwithstanding disadvantages in gate fidelity and operation time, leading companies such as ColdQuanta and Pasqal believe that cold atom technology could be advantaged in horizontal scaling using fiber optics (infrared light) and in the long term could even offer a memory scheme for quantum computers, known as QRAM.
Each of the primary technologies and the companies pursuing them have significant advantages, including deep funding pockets and expanding ecosystems of partners, suppliers, and customers. But the jury remains out on which technology will win the race, as each continues to experience distinct challenges relating to qubit quality, connectivity and scale. (See Exhibit 6.)
Some partners and customers are hedging their quantum bets by playing in more than one technology ecosystem at the same time. (See “How Goldman Sachs Stays at the Front Edge of the Quantum Computing Curve.”)
How Goldman Sachs Stays at the Front Edge of the Quantum Computing Curve
The next step is identifying the problems that would be implemented on real hardware and—crucially—when. Zeng’s team is developing some algorithms internally, but nearly all of its research is conducted in partnership in a resolutely non-exclusive approach. “Our primary goal is to find the right experts who complement the skills we have internally, whether that’s at a particular startup, a hardware player, or in academia,” Zeng said. “But we also give some consideration to distributing our findings. The field is still young, and we all have a role to play in advancing it.”
A large share of what Goldman Sachs has developed in partnership has been made public. In late 2020, Goldman and IBM published their research into a “new approach that dramatically cuts the resource requirements for pricing financial derivatives using quantum
Dividing the Quantum Computing Pie
Of the $450 billion to $850 billion in value that we expect to be created by quantum computing at full-scale fault tolerance, about 80% ($360 billion-$680 billion) should accrue to end users, such as biopharma and financial services companies, with the remainder ($90 billion-$170 billion) flowing to quantum computing industry players. (See Exhibit 7.)
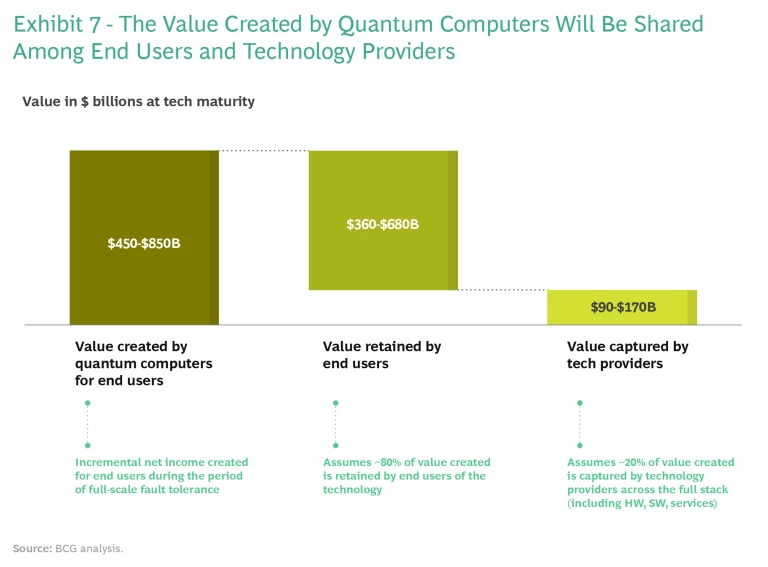
Within the quantum computing stack, about 50% of the market is expected to accrue to hardware providers in the early stages of the technology’s maturity, before value is more evenly shared with software, professional services, and networking companies over time. The key constraint in the industry is the availability of sufficiently powerful hardware. We therefore expect demand to outstrip supply when good hardware is developed. This is the pattern that developed with classical computers. In 1975, the year Microsoft was founded, hardware commanded more than 80% of the ICT market versus 25% today, when it is considered largely a commodity.
Investors are betting on quantum computing following a similar course: about 70% of today’s equity investments have been in hardware, where the major technological and engineering roadblocks to commercialization need to be surmounted in the near term. Key engineering challenges include scalability (interconnecting qubits and systems), stability (error correction and control systems), and operations (hybrid architectures that interface with classical computing).
Revenues for commercial research in quantum computing in 2020 exceeded $300 million, a number that is growing fast today as confidence in the technology increases. The total market is expected to explode once quantum advantage is reached. Some believe this could come as soon as 2023 to 2025. Once quantum advantage is established and actual applications reach the market, value for the industry and customers will expand rapidly, surpassing $1 billion during the NISQ era and exceeding $90 billion during the period of full-scale fault tolerance. (See Exhibit 8.)
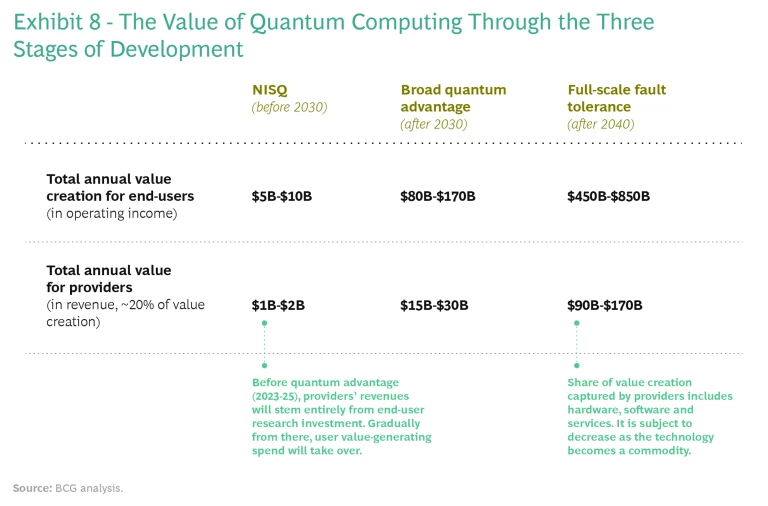
How to Play
Perhaps no previous technology has generated as much enthusiasm with as little certainty around how it ultimately will be fabricated. This enthusiasm is not misguided, in our opinion. Tech providers, end users and potential investors need to determine how they want to play and what they can do to get ready. The answer varies for each type of player. (See Exhibit 9.)
What Happens When If Turns to When in Quantum Computing
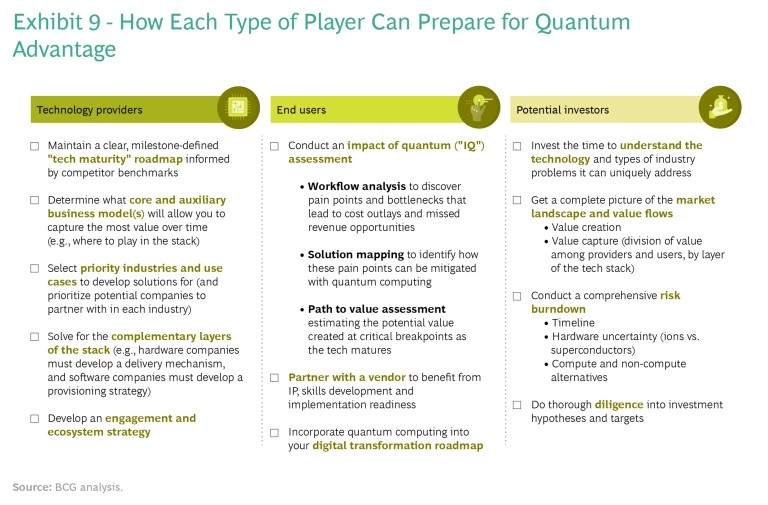
Tech Providers. Technology companies, especially hardware makers, should develop (or maintain) a clear milestone-defined quantum maturity roadmap informed by competitor benchmarks and intelligence. They can determine the business model(s) that will allow them to capture the most value over time—where they should play in the quantum computing stack and solving for complementary layers of the stack. Hardware companies will likely develop delivery mechanisms, for example, while software providers develop provisioning strategies. All will want to develop an engagement and ecosystem strategy and prioritize the industries, use cases, and potential partners in each sector where they see the greatest opportunities for value. These opportunities will evolve over time based on the actions of other players, so early movers will have the most open playing field.
End Users. Companies in multiple industries likely to benefit from quantum computing should start now with an impact of quantum (IQ) assessment. It will map potentially quantum-advantaged solutions to issues or processes in their businesses (portfolio optimization in finance, for example, or simulated design in industrial engineering). They also should assess the value and costs associated with building a quantum capability. Depending on the path to value and the timeline, end users may benefit from the IP, skills development, and implementation readiness that come from partnering with a tech provider. Companies in affected industries should also incorporate quantum computing into their digital transformation roadmaps.
We encourage investors to educate themselves in three areas: technology, value flow, and risk.
Investors. We encourage investors to educate themselves in three areas: technology, value flow, and risk. The first includes investing the time to understand the types of industry problems quantum computing can best address, building an understanding of the five key technologies being developed, and staying current on the outlook for each. It may make sense to hedge with investments in more than one technology camp. Analysis should include the division of value between providers and users as well as among the layers of the stack. Risks include likelihood of success and, importantly, the timeline to incremental value delivery, especially in the context of hardware uncertainties. Topical complexity will necessitate thorough diligence on all investment hypotheses and targets.
Tech + Us: Monthly insights for harnessing the full potential of AI and tech.
One thing is clear for all potential players in quantum computing: no one can afford to sit on the sidelines any longer while competitors snap up IP, talent, and ecosystem relationships. Even if there remains a long road to the finish line, the field is hurtling toward a number of important milestones. We expect early movers to build the kind of lead that lasts.
The authors are grateful to Flora Muniz-Lovas and Chris Dingus for their assistance in preparing this report.