Despite their best intentions, the path to data maturity for many companies in asset-intensive industries has been bumpy. Companies in the energy, automotive, and industrial goods sectors, for example, scored at the bottom of our 2021 BCG Data Capability Maturity Assessment survey .
The reasons for this vary from complex legacy IT systems and longstanding organizational and data siloes to legitimate regulatory, financial, and cybersecurity concerns. But the impacts are the same—inefficient operations, poor asset and capacity utilization, and lower margins, among others.
The potential benefits of data and advanced analytics for asset-intensive companies are many: reduced capex, improved operational efficiency, greater asset utilization, and more. Early adopters are already creating digital twins of their supply chains to optimize production and utilization and improving margins by developing dynamic maintenance scheduling of assets based on failure prediction analysis.
The potential benefits of data and advanced analytics for asset-intensive companies are many: reduced capex, improved operational efficiency, greater asset utilization, and more.
In what follows, we examine the challenges asset-intensive companies face in their efforts to apply data centricity to assets and operations and offer solutions to overcoming these challenges. To this end, we outline the different dimensions required for a successful data transformation. What we call the “ flywheel approach ” to data maturity is designed to enable companies to build the capabilities needed over time, making sure they reinforce each other and deliver real business value throughout the program.
How Early Adopters Are Benefiting
There is clear evidence that increased data and digital maturity leads to better performance. Our research into bionic companies shows that they have 1.8 times higher EBITDA growth, spend 1.5 times more on R&D, and achieve 2.4 times greater enterprise value growth, on average, than do digital laggards.
Early adopters in asset-intensive industries are differentiating themselves through data. For example, aircraft engine manufacturer Rolls-Royce’s innovation lab has developed an intelligent borescope, leveraging computer vision and artificial intelligence (AI) to reduce engine inspection times by as much as 75%, resulting in potential savings of up to $100 million over five years. In 2021, Norwegian energy producer Equinor launched a partnership with Cognite, an industrial data analytics provider, to accelerate its data ambitions. And Rio Tinto, a major global mining company, is using predictive maintenance on their autonomous trucks, each equipped with 45 sensors that generate 5 terabytes of data daily, to extend their technical life by over 20%.
Given such positive results, it behooves all asset-intensive companies to work toward capturing the benefits of data.
Common Obstacles to Data Maturity
There are three primary reasons asset-intensive companies lag in their data maturity efforts: entrenched organizational silos, a focus on IT costs, and the very nature of asset-intensive industries.
Organizational Siloes. Asset-intensive companies have long been structured around individual business units—separate siloed entities focused on specific objectives—and years of M&A activity have only exacerbated their complexity. Typically, each business unit has maintained its own IT systems, hampering the ability to share data across the organization.
Focus on IT Costs. These companies also tend to focus their capex plans on their legacy physical assets rather than on IT, which is typically not considered core to the business. And because IT management efforts have focused on reducing costs rather than adding business value, many companies still depend on complex legacy IT systems. Some of these systems date back to the 1960s and 1970s and must be maintained to support historical assets or for regulatory reasons (in the case of aerospace engineering companies, for example). These complex legacy IT systems make it very difficult to access and collect needed data efficiently.
Historical optimization for cost has also created a heavy dependence on outsourcing and long-term software vendor contracts that reduce costs but do not reward vendors for innovation. And hard experience with unsuccessful IT simplification and integration projects only lowers the appetite to invest in major data transformation projects.
Nature of the Industry. Most companies in asset-intensive industries are primarily B2B focused. As a result, they feel less need to use data to generate a better understanding of their customers’ needs or to develop the kinds of digitally enhanced, data-dependent marketing efforts now common among B2C companies. Security concerns also mitigate against the need to improve data maturity. Companies in some industries—aerospace and defense, for example—have grave concerns about the cybersecurity risks and regulatory issues surrounding greater data integration and transparency.
Furthermore, many highly regulated asset-intensive companies are required to maintain high levels of operational resilience, which often means keeping their old IT architecture, systems, and data as a fail-safe even after new data systems have been implemented. Grid network operators, for example, must preserve their backup systems to ensure that the grid continues to function in the event of a loss of connectivity with newer implementations.
Shortcomings in Data Transformation Execution
Still, numerous asset-intensive companies have made efforts to transform themselves into data-centric organizations. All too many of these programs, however, fall short of their objective due to over-indexing on one aspect of the solution or another. This typically takes three forms:
Tech for Tech’s Sake. Companies often face a chicken-or-egg problem in trying to become data centric: business functions aren’t looking to implement use cases because the technology is not ready, while IT does not prioritize getting the technology in place due to lack of demand. IT will often take the lead in breaking this cycle using a “build it and they will come” mentality. Despite their best efforts, however, many of these IT-led transformations work in the assumption that business users will benefit from it, but without working with the business to create what’s really needed. This outcome is often associated with the wholesale replacement of legacy tools and systems. But without input from the business, such efforts are likely to lead to even greater inefficiency and frustration on the part of business users, and the investments will all too often be wasted.
For example, an automotive original equipment manufacturer invested in a large-scale data platform designed to support its overall data transformation journey. The platform was ready to be used, on a technical level, but the company had not yet designed and implemented the corresponding operating model for how the business would use it, or trained employees in the capabilities needed to generate the use cases that would add real business value.
Siloed Implementation. Here, a company might digitize a part of a single business function, such as supply chain management or human resources, developing specific use cases to demonstrate the potential of better data management and the art of the possible. All too often, however, such efforts ignore the bigger picture, missing opportunities to reuse individual components of the use case in the next wave of digitization across other units in the company. Each use case is therefore supported by its own relevant point solutions and implemented within the silo, without putting the necessary scaling capabilities in place. As a result, the required data and technology, modeled and structured to the individual use case, is not reusable across the organization.
An electrical grid operator, for example, might run a pilot program to track and manage the vegetation surrounding its lines. This would likely require several types of data, including historical weather data, vegetation data, satellite imagery, grid construction data, and field service skills and work schedules, which could be useful to other parts of the company, such as HR or grid construction. And the technology capabilities needed, such as data capture, storage, management, and analytics, are equally key. But the implementation is of minimal value if it cannot be leveraged across the company.
Poor Data Quality. Companies are often well aware that the quality of their data—the raw material for delivering value—is insufficient, and so launch a data governance initiative to address it. Yet the effort to improve their data governance is often limited to appointing people in standard data owner and custodian roles and providing an assortment of data management tools. These initiatives tend to fall short due to a lack of focus on how to structure and model the company’s data, insufficient training and change management, and an implementation approach that does not focus on capturing the maximum business value from the effort.
For example, an international chemical company was looking to use its data to optimize its pricing strategy with the help of AI. As part of the implementation, the company limited its new data governance effort to appointing business users to newly defined roles, such as data owners. As a result of insufficient training, skills, and clarity as to the scope and business value of the project, however, the company soon abandoned the effort to incorporate AI into pricing and returned to the status quo.
Data transformation projects struggle when there is no companywide organizational structure driving a multidimensional approach. As a result, use cases cannot be scaled across the company, IT departments either remain “order takers” for the business, focus their efforts on data governance issues, or make technology choices with little regard for the needs of the business. The result: new IT platforms and new business capabilities that don’t create any real business value.
Six Dimensions of Data Transformation
Only by embarking on a holistic transformation of their data collection, management, and analysis capabilities can asset-intensive companies reap the full benefits of data centricity. In doing so, it is critical that companies take all six transformation dimensions into account, without which the chances of success will be significantly decreased.
- Vision. Articulate the overall ambition, how it synchs with the organization’s overall strategy and the business value to be gained. Determine whether the goal is to improve current practices or carry out a radical transformation and clarify the specifics across each of the dimension below.
- Use Cases. Set out the transformation’s desired business outcomes and the main use cases for achieving them. Define the physical assets to focus on, how better data will increase their value, and by how much. Decide how best to sequence the use cases to reinforce and build up maturity in the other dimensions.
- Analytics Setup. Determine which analytics functions to implement, ensuring that they will lead to concrete results. And critically, make sure you have the talent in place needed to implement them—and to deliver on the other dimensions of the transformation.
- Data Governance. Put in place the organization and people needed to carry out proper data management. Ensure that the proposed use cases have the quality data needed, by setting up the governance mechanisms needed to augment and manage data quality together with the business and IT functions. Governance mechanisms should cover data structures, quality, policies, processes, and tools.
- Data Infrastructure. Determine and implement the proper data infrastructure and platform capabilities suitable to the vision and proposed use cases, including the right operating model and data technologies. Ensure that it incorporates end-to-end processes, from the capture of multiple data sources to the execution of the use cases at scale.
- Data Ecosystem. Define the external parties with which to engage in carrying out the transformation and the data sources needed. Work out what your company’s role will be in managing the ecosystem.
Key to each of the dimensions above is change management. Only by embedding the new data-centric behavior into the organization and across all the dimensions of the transformation will the shift from dependence on legacy systems to becoming a data-driven company be successful. Company leaders would be wise to consider implementing the many new ways of working that have proved successful in helping organizations carry out complex data transformations.
Companies should not try to implement each of these dimensions sequentially. Instead, they should adopt what we call a “ flywheel approach .” This encourages companies to think big but start small. Begin by clarifying the overall vision, and at the same time, develop specific business-oriented use cases while building out and delivering the data and analytics capabilities required for each use case on a just-in-time basis. In parallel, companies should develop and implement a thorough change management program in parallel with the implementation of early use cases.
Subscribe to our Digital, Technology, and Data E-Alert.
What will differentiate successful companies from the rest will be their ability to implement a flywheel approach while aligning data roadmaps across the organization to identify synergies among them and maintaining a company-wide view of all data initiatives.
The challenge lies in managing competing objectives—delivering business value quickly while at the same time developing reusable components for gathering and managing data across the company. If applied correctly, the flywheel approach can be very effective in creating business value early in the process—value that can subsequently help to fund the ongoing transformation journey.
Building Asset Journeys
Much like the concept of customer journeys, which have become common practice in B2C-focused industries, asset-intensive companies should build out “asset journeys,” defining use cases across the lifecycle of each asset rather than through the companies’ organizational structures and functions. The journey goes from the planning stage, where the asset’s purpose and value are determined, and continues through design and construction, operation, maintenance and decommissioning and replacement. (See the exhibit.) This approach will allow companies to identify the data and use cases needed for each asset throughout its lifecycle and across all organizational siloes involved.
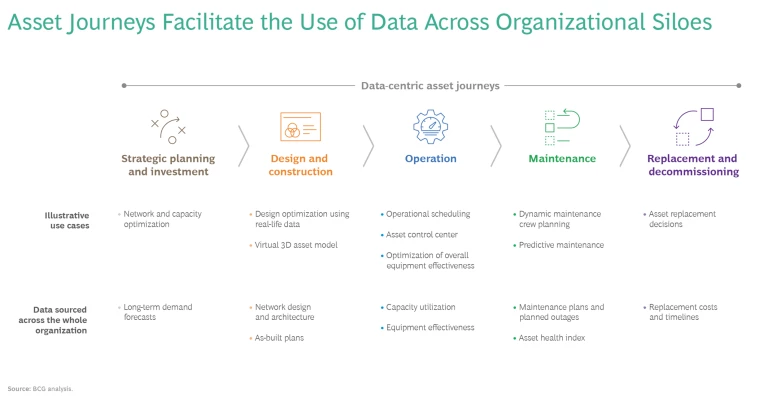
Asset-intensive companies should start small, zooming in on a particular stage of the asset journey based on a prioritization of the effort required to develop the relevant data use case and its expected value. The low-hanging fruit can often be found in improving the operational efficiency of a particular asset—its overall equipment efficiency and capacity utilization. Once that is achieved, they can then scale up the new use case to other assets while developing more complex use cases pertinent to that same asset. These typically require the collection and analysis of data across longer time horizons and from different areas of the organization, such as leveraging asset failure data to improve the design of new assets.
If interest rates keep rising, inflation remains high, and the global economy slows down, the downward pressure on margins in asset-intensive industries will only increase, increasing the difficulty of financing large-scale capital projects. Under such conditions, improved operational efficiency and greater asset utilization will be critical for competitive advantage. This in turn will be highly dependent on the effectiveness of asset-intensive companies’ data collection and management processes.
Most of these companies are fully aware of the value of data maturity, but the task can seem daunting, given their dependence on long-standing, heavily siloed legacy systems. That’s why applying the flywheel approach across all six dimensions is the key to success. Now is the time to start turning the flywheel.