A lot has happened since 2021, when we published our forecast for the quantum computing market. Both the new technology and its classical computing alternative have advanced in unforeseen ways, changing the trajectory, if not the overall direction, of the developing market. It’s time to update our analysis.
Where Quantum Computing Stands Versus Its Potential and the Competition
The key question is whether quantum computing is finally nearing a point where it can fulfill its transformative potential. The answer, right now, is mixed.
Challenges persist. Quantum computing today provides no tangible advantage over classical computing in either commercial or scientific applications. Though experts agree that there are clear scientific and commercial problems for which quantum solutions will one day far surpass the classical alternative, the newer technology has yet to demonstrate this advantage at scale. Fidelity—the accuracy of quantum operations—is still not up to par and hinders broader adoption. Meanwhile, classical computing continues to raise the bar thanks to the big strides it has taken in hardware (such as GPUs), algorithms, and artificial intelligence (AI) libraries and frameworks.
At the same time, quantum is showing undeniable momentum. The number of physical qubits on a quantum circuit—a key indicator of computing capability—has been doubling every one to two years since 2018, reflecting significant technological progress. This trend is expected to continue for at least the next three to five years.
Despite a 50% drop in overall tech investments, quantum computing attracted $1.2 billion from venture capitalists in 2023, underscoring continued investor confidence in its future.
Governments around the world are active in this area, too. Led by the US and China, they have been making big investments in the technology, envisioning a future in which quantum computing plays a central role in national security and economic growth. Public sector support is likely to exceed $10 billion over the next three to five years, giving the technology enough runway to scale.
This article navigates these contrasting narratives, examining how the persistent hurdles and burgeoning advances could coalesce to unleash quantum computing’s potential.
The Challenges of Value Creation in the NISQ Era
Three years ago, we expected the market to mature in three phases, and this is still the case: noisy intermediate-scale quantum (NISQ), until 2030; broad quantum advantage, 2030–2040; and full-scale fault tolerance, after 2040. (See Exhibit 1.)
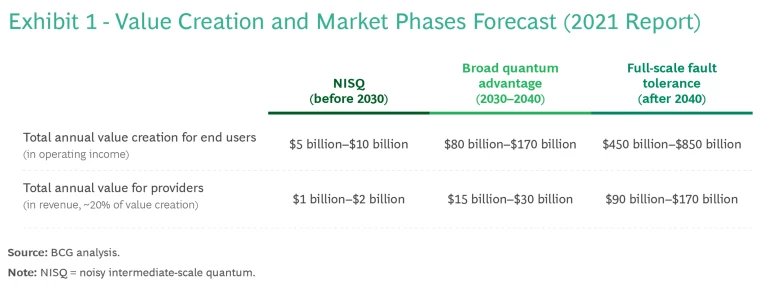
We also remain confident about our projection that quantum computing will create $450 billion to $850 billion of economic value, sustaining a market in the range of $90 billion to $170 billion for hardware and software providers by 2040. (This projection is consistent with the projected market for high-powered computer providers, which is expected to reach $125 billion by 2040 according to Statista.)
Our assumptions for near-term value creation in the NISQ era have proved optimistic, however, and require revision. Accordingly, some companies in some industries will need to rethink how they approach quantum technology.
We based our initial projections on two key criteria that we assessed would lead hardware and software advances to converge at a point where they could then surpass the performance of classical computing:
- Hardware Performance. We expected quantum volume (as measured by the number of qubits and their fidelity) to double every one to two years.
- Software Performance. We anticipated that new quantum algorithms would be developed to harness quantum hardware better and accelerate benefits (such as by increasing speed, improving the efficiency of energy usage, and unlocking new uses) over classical computing in affected use cases.
For our 2021 forecast, we assessed more than 100 use cases stemming from four types of computational problems where quantum could have a technological advantage: simulation, optimization, machine learning, and cryptography. (See Exhibit 2.)
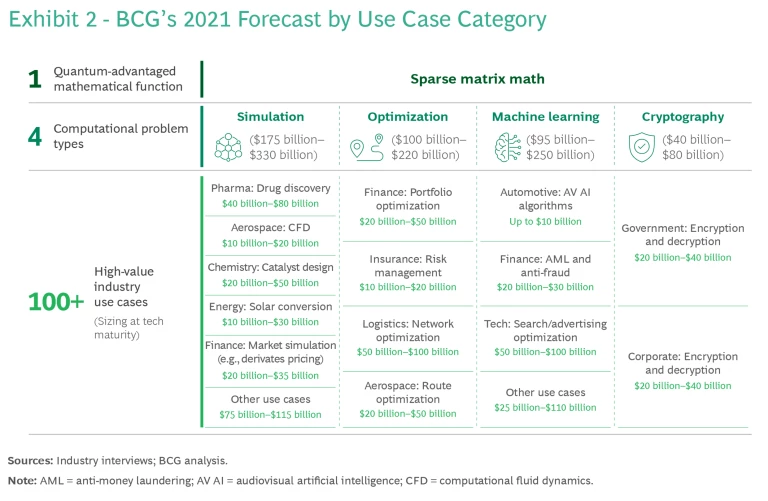
In our assessment, we assumed that developers and users would pursue three types of use cases: optimal, intractable, and brand new.
Optimal. For many complex optimization, simulation, and machine-learning problems, classical computers employ heuristic approaches to find solutions that typically fall within a 5% to 20% margin of error of the optimal answer. Many experts expected near-term quantum solutions to narrow that margin with better accuracy and to deliver their solutions more quickly.
Unfortunately, NISQ algorithms (such as the quantum approximate optimization algorithm and the variational quantum eigensolver) also rely on heuristic methods, with greater inherent uncertainty than classical solvers, which benefit from years and even decades of experience and hardware improvements. Classical solvers and AI algorithms are likely to outperform quantum computing on most intractable problems until error correction can decisively demonstrate advantage.
Intractable. Quantum computers can solve some problems that are beyond the capabilities of classical computers. As the size of these “intractable” problems increases, the time and computational resources required to solve them exactly (as opposed to approximately) grow exponentially, making them unmanageable for classical machines. Examples include computing the color emitted by a dye, the conductivity of a material, and the properties of a molecule in a drug. For practical systems, exact calculations require more transistors than there are atoms in the universe—which is why Richard Feynman proposed quantum computing in 1981.
The word exact (with regard to both accuracy and precision) is important. AI has made inroads into problems considered intractable at the time of our 2021 publication, and this has led to speculation that quantum computing may have a lesser role to play in tackling these problems because AI solutions could offer a “good enough” alternative. The reality is more nuanced. AI relies on learning solutions from data (such as in the case of large language models, which learn from semantic relationships in existing language and data). The availability and quality of training data sets impose inherent limitations on the accuracy and precision of AI answers. Quantum computing does not suffer from such constraints and thus offers superior capabilities.
By design, AI faces two major limitations: the results contain a certain degree of error, and they lose accuracy as they venture further from the training data set. In the large-molecule universe, including proteins, which is thought to be in the 1050 range, we can assume there will never be enough training data to represent the possibilities faithfully. Quantum computing will likely remain the best solution for today’s intractable problems, notably by simulating nature as Feynman envisioned.
Brand New. These are use cases that we don’t know we will need yet, in the same way that ridesharing became obvious and all but indispensable once smartphones made it possible. Our 2021 and current market forecasts estimate that brand new use cases will generate 20% to 30% of future value, based on prior introductions of new technology .
Hardware and Software Fall Short
The NISQ era has not lived up to our expectations for two reasons. First, technical hurdles in hardware development are proving tough to overcome. Qubit numbers have been increasing fast, but fidelity remains a big issue. Analysis by the community-driven platform Metriq, which tracks quantum technology benchmarks, suggests that with existing software, most valuable use cases require both 10,000 to 20,000 qubit-gate operations and close to 100% gate fidelity. But circuits of more than 30 qubits have so far achieved at best a 99.5% fidelity rate (a barrier that was only partially broken in April 2024 when collaborative efforts by Microsoft and Quantinuum on their H1 systems reached a "three nines" rate for 2-qubit gates). Because errors accumulate exponentially, even the best hardware fails after about 1,000 to 10,000 qubit-gate operations. Useful algorithms need millions of gate operations (even billions in the case of Shor’s algorithm), so quantum machines still need to improve by many orders of magnitude.
Development of new algorithms has lagged, too. In fact, most significant algorithm advances were formalized between the 1980s and the 2010s. Little progress has occurred in the past ten years. (See Exhibit 3.)
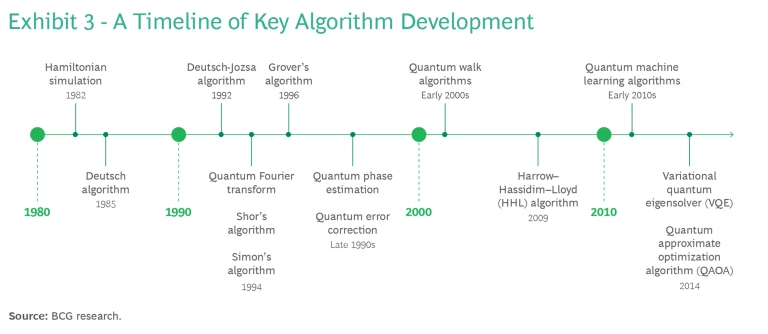
Second, competition from classical computing has been fiercer than we anticipated. In particular, AI has exceeded expectations in scientific fields, offering viable alternatives for previously intractable problems. In the long run, quantum computing will bring definitive advantages to handling highly complex problems—such as many-body physics and NP-hard optimization—over classical solvers, which rely on imperfect heuristic and approximate algorithms. NISQ limitations force current quantum algorithms to depend on heuristic approaches too, and this reliance makes advantage impossible to prove consistently, if there is any.
Together, these factors have prevented quantum computing from realizing a definitive advantage over classical systems in both hardware and software capabilities, at least through the approach based on digital gates. There are alternatives. Companies such as D-Wave, Pasqal, Kipu Quantum, and Qilimanjaro have chosen to pursue analog and hybrid (analog combined with few gates) quantum computing. These approaches could unlock commercial use cases in the short term without needing too many gate operations.
Recent advances confirm that quantum computing continues to achieve significant progress. Innovative prototypes have demonstrated substantial improvements in solving complex problems, showcasing the potential for optimization, quantum simulations, and material simulations. The development of application-specific quantum chips and the expansion of cloud-based quantum computing services highlight the movement toward delivering practical and scalable quantum solutions.
The Impact on Providers
By leveraging analog methodologies, quantum machines can still deliver tangible near-term value, especially in materials and chemicals simulations, ranging from $100 million to $500 million a year during the NISQ era. Although this forecast is significantly lower than our previous projection, we do not expect it to have a major impact on the market for hardware and software providers. We continue to foresee a provider market in the range of $1 billion to $2 billion by 2030, in line with projections by others.
Three factors are in play here. First, as it has done in the past with technologies such as semiconductors, the internet, and GPS, the public sector is providing substantial support through orders and grants. For example, the UK Ministry of Defence has purchased quantum computers from ORCA Computing even though these machines do not yet the break the 40-qubit threshold that a classical high-powered computer can simulate. We estimate that public orders of quantum computers already support more than half of the market. Given existing program announcements and the geopolitical importance of quantum technologies, this level of demand should persist over the next three to five years. (See Exhibit 4.)
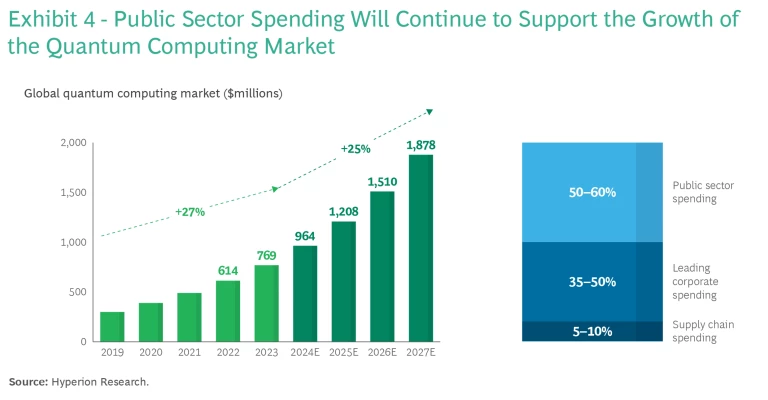
Second, leading corporations are investing in enterprise-grade quantum capabilities. In a 2023 publication on quantum adoption , we tracked more than 100 active proof-of-concept projects among Fortune 500 companies, representing a total investment of about $300 million. These companies have ambitions to become first movers—for example, by filing patents for promising materials discovered with quantum computers or by devising hedging strategies that more rapidly exploit market imperfections. Despite AI advances and NISQ setbacks, we expect more companies to pursue long-term programs in the coming years, thanks to the tangible prospects for technological advances, notably in error correction, and to use of AI as a source of training data with little overlap in use cases.
Third, providers can generate revenues by forming supply chains that involve such equipment as controls, dilution fridges, lasers, vacuums, and software. We estimate that supply chain spending will account for 5% to 10% of quantum computing hardware and software revenues this year.
Error Correction Will Accelerate Progress
The prospects for qubit error correction were theoretical and uncertain in 2021. Most experts predicted achievement of this milestone sometime after 2030; some said that it would never materialize. But the past three years have seen substantial practical advances. A collaboration among Harvard, QuEra, MIT, and NIST/UMD demonstrated error correction with 48 logical qubits on the neutral atoms platform. Superconducting qubit leader IBM created another innovative error-correcting code—one that is ten times more efficient than prior methods. Recently, Microsoft and Quantinuum demonstrated an 800-times error reduction with trapped ions. Other clever innovations such as stronger hardware encoding (by the quantum design firm Alice & Bob) have also come into play since 2021, fostering growing optimism about the practicality of error correction.
In our 2021 forecast, we noted that 90% of the total calculated value was contingent on improvement in error correction. Public roadmaps from IBM, QuEra, and Alice & Bob, among others, promise to achieve full error correction by 2029 at the latest. Its sooner-than-expected arrival will accelerate time-to-value for end users in the medium term.
Enterprise Impact
The bottom line is that while quantum computing remains an exciting prospect in some sectors, the technology remains in its infancy and offers limited immediate value for most enterprises. Not all industries will want to embark on the quantum journey. Investment is most clearly justifiable for companies that can capitalize on significant gains as soon as error correction improves (through transformative advances in molecular development, for example) or that seek a first-mover advantage (such as quantum computing services provided by tech giants or cybersecurity enhancement through new decryption methods).
Five industries (followed by the public sector) top the list of those positioned to benefit from error-corrected quantum computing. The technology provides each with its own set of benefits:
- Technology companies need to stay ahead in tech race, and being first (or early) to provide quantum computing and hybrid tech services will put tech providers in a strong competitive position, potentially for decades to come.
- Chemicals and agricultural companies will find quantum computing a powerful tool to enhance their molecular modeling and simulation capabilities, leading to breakthroughs in material science and crop protection.
- Pharmaceuticals companies will be able to model complex molecular interactions at unprecedented speeds, accelerating drug discovery and reducing time-to-market for new medications.
- The defense and space industry will achieve significant advances in secure communications, complex system simulations, and other technologies critical for national security and advanced aerospace projects.
- Financial institutions will gain the ability to process vast amounts of data for risk assessment and portfolio optimization, providing a competitive edge in a fast-paced market.
Shifting Focus
There is a strong focus today on enabling manufacturers to increase the number of qubits in a quantum processor. This is undeniably key in the quantum race. Still, we expect the focus of development to broaden to encompass additional criteria as users seek to fully harness emerging value. (See Exhibit 5).
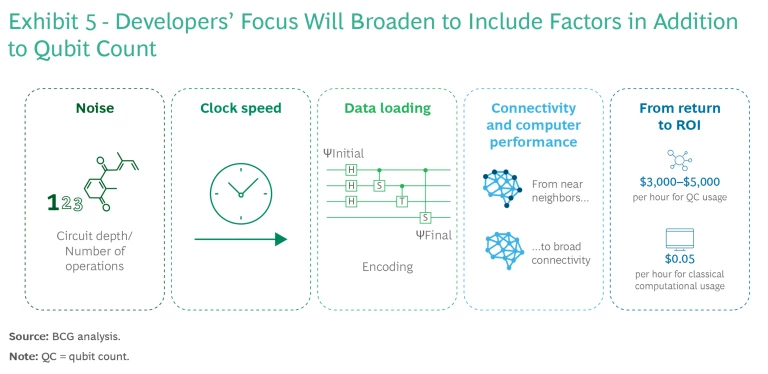
Noise. As mentioned earlier, low fidelity rates prevent NISQ-era algorithms from providing real ROI in the near term. At least in the case of digital computing, it will be necessary to implement error correction code to drastically reduce noise and permit increased circuit depth.
Clock Speed. Speed is another variable that may need to improve, since quantum computers today are slow compared with their classical counterparts. There is a big difference even among the different quantum computing modalities. For example, the current state-of-the-art performance level for cold atom systems is thought to be in the kHz range, but we expect superconducting qubits to reach clock speeds in the MHz range. Slower operational speeds impose severe limitations on practical applications. According to a 2006 study on the architecture-dependent execution time of Shor’s algorithm, factoring a 576-bit number within a month requires a clock rate of 4 kHz, while a clock rate of 1 MHz could complete the task in about three hours. As algorithms emerge that provide exponential advantage over classical methods, quantum computing’s clock speed limitations will diminish.
Data Loading. The ability to load data into a quantum computer is a key bottleneck today. Solving a computational problem typically starts with encoding the information into the bits of the computer. The problem is that qubits are scarce. Smart algorithms can map the data in the computer with a minimum of requirements, but quantum machines are far from competing with the terabytes of processing power available in classical computers. An alternative approach that avoids loading classical data would be to compute directly from a native quantum state—for instance, with data coming from a previous computation or from quantum sensors.
Connectivity and Computer Performance. Defined as the ability to apply gates between distant qubits, connectivity is crucial for maximizing the speed and efficiency of quantum algorithms. Therefore, it will play a significant role in a quantum architecture’s ability to implement efficient error correction code. Some quantum technologies, such as trapped ions and neutral atoms, already benefit from high intrinsic connectivity, but alternative technologies, such as superconducting and silicon qubits, are usually limited to near-neighbor connectivity (linking only with adjacent qubits) and so experience computational overhead (by moving qubits around the circuit at every step).
From Return to ROI. The focus of financial assessments will shift over time from expectations for long-term return to near-term ROI. Quantum computing is currently 100,000 times more expensive per hour than classical computing ($1,000 to $5,000 per hour for quantum machines compared with $0.05 per hour for classical computing), but we expect this gap to shrink with increasing scale over time. While this comparison does not account for qualitative differences in outcomes, it does highlight that not all use cases will derive sufficient value from quantum computing to justify the cost. Moreover, corporate buyers typically aim for a one-year break-even point—although a three- to five-year break-even target may be acceptable in certain cases. In this scenario, the most favored use cases for quantum technologies will be those that promise a rapid ROI.
The More Things Change…
A lot has indeed changed since 2021. Perhaps the most remarkable thing, though, is the stability of the overall picture. Impediments to quantum computing in the near term, such as circuit depth and fidelity rates, do not threaten the long-term development of the technology or the market. The challenges of 2021 are still the challenges today, and they are closer to resolution. Quantum computing is still on track to create enormous value in sectors where it can solve business problems faster or better. End users need to build out their partnerships with providers and develop their own skill sets because today as in 2021 quantum computing remains a winner-take-most technology.
The authors would like to thank Laurent Prost and Elie Gouzien from Alice & Bob and Helmut Katzgraber from AWS for their help and support in the production of this paper.