Artificial intelligence and machine learning (AI/ML) passed a milestone in 2024 when the total number of medtech products to receive FDA clearance exceeded 1,000. (See Exhibit 1.) Impressively, over 100 of these AI/ML products received a Breakthrough Device designation, entitling them to priority FDA review, flexible clinical trial design, enhanced regulatory interaction, and first mover advantage as they hit the market. AI/ML-enabled medtech products tend to serve at the frontlines of health care, offering real-time and highly personalized solutions for clinical care and decision making.
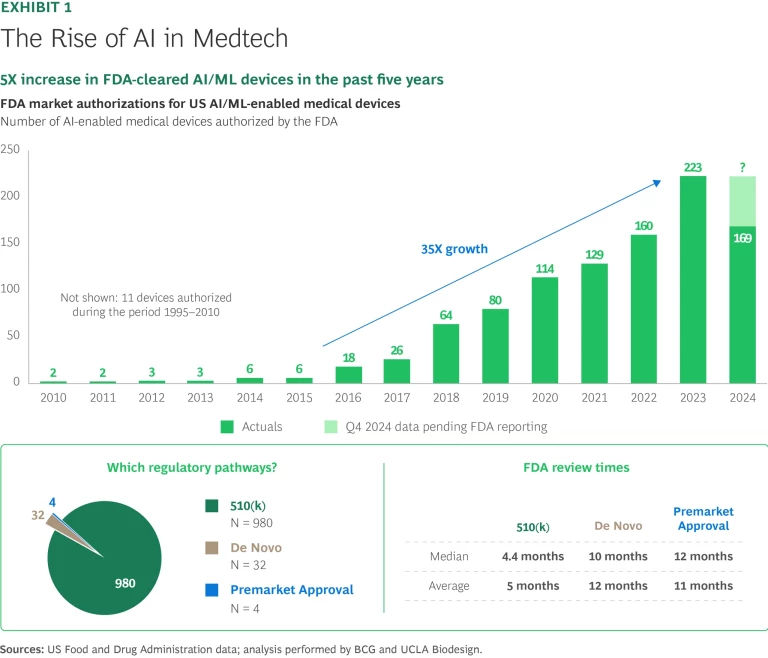
Software the New Battleground
Software as a medical device (SaMD) is a key element in the medtech AI/ML boom, representing 699 of the 980 510k and 22 of the 32 De Novo clearances in the past five years. So far, radiology has garnered the most AI/ML device clearances among medical specialties, accounting for about 70% of the total. However, the versatility of AI-powered software seems likely to change this disproportionate representation. A new report by a joint team from BCG and UCLA Biodesign identified six distinct patterns within the AI/ML surge, ranging from products that expedite review time and enhance detection via automated image analysis to products that extract and combine decentralized inputs from wearables and smartphones to help classify disease states. Health care providers are putting AI/ML-enabled devices to work across nearly all specialties, with the most significant gains to date in cardiology.
Given the prominence of software in the AI/ML gold rush, new strike zones have emerged alongside others long known as medtech innovation hubs. Not surprisingly, the US accounts for half of the approved devices, with California (including a notable concentration in the San Francisco Bay Area) yielding the largest US regional share and 13% of the global total. Other US centers—in Wisconsin, Pennsylvania, and Tennessee—owe their prominence to hosting the US headquarters of some of the world’s largest diagnostic imaging companies. Outside the US, Tel Aviv has delivered 8% of the global tally of FDA-approved medtech products, and Paris, Shanghai, Seoul, and Tokyo collectively account for about 20%.
AI/ML Devices: Over 1,000 FDA-Cleared Products
The BCG/UCLA Biodesign team evaluated a sample of 691 AI/ML devices (out of a total of 1,016) that gained FDA 510(k) clearance from 2010 to 2024, comparing their time to approval with that of 6,404 comparable standard medtech products cleared during the same time period. The team found that the median FDA clearance time is longer for AI/ML-enabled prospects (133 days) than for standard medtech devices (106 days). Medtech executives and FDA sources point to the ongoing learning curve associated with SaMD, as well as to persistent shortages of FDA staff equipped to regulate software products. In comparison to standard medical devices, AI/ML-enabled products seem to benefit less from augmenting FDA staff with third-party reviewers. Whereas clearance times for traditional medtech offerings plunge from a median of 114 days to just 29 days under third-party review, outside reviewers shave just 19 days (from 134 to 115) off AI/ML median time to approval.
Regulatory Shades of Gray
Despite the surge in FDA clearances for AI/ML-enabled products, questions linger about how to incorporate adaptive logic and
generative AI
(GenAI) into medtech products. The FDA requires medtech companies to file a predetermined change control plan (PCCP) for AI/ML products that use adaptive logic—a learning algorithm that draws on updated, real-world data rather than depending on training data alone to derive refined solutions. As of Q3 2024, only three such products had been approved. The climb appears steeper yet for GenAI technology, which creates original content on the basis of its training guardrails and user input prompts. No GenAI products have received FDA clearance yet. One promising area, however, involves using GenAI to extrapolate training data sets for medtech AI products from de-identified clinical data. If successful, this approach could help GenAI products sidestep thorny data privacy and ownership issues, although it would also raise the need for new technical and regulatory skills to set operational boundaries and uphold patient safety.
$14 Billion in VC Investment
In a BCG/UCLA Biodesign survey, 68% of medtech executives expressed a high level of optimism about their ability to win approval for their AI/ML offerings, but somewhat less confidence (50% highly or moderately optimistic) about their prospects for successfully commercializing those products. (See Exhibit 2.) For venture capitalists (VCs) staking their medtech claims on AI/ML-enabled products, however, confidence reigns supreme. A review of Pitchbook records indicates that 3,057 identifiable VCs have plowed $14 billion into 387 AI/ML enterprises since 2010. Funding peaked in the period from 2020 to 2022 before falling off in 2023 as US interest rates moved sharply higher.
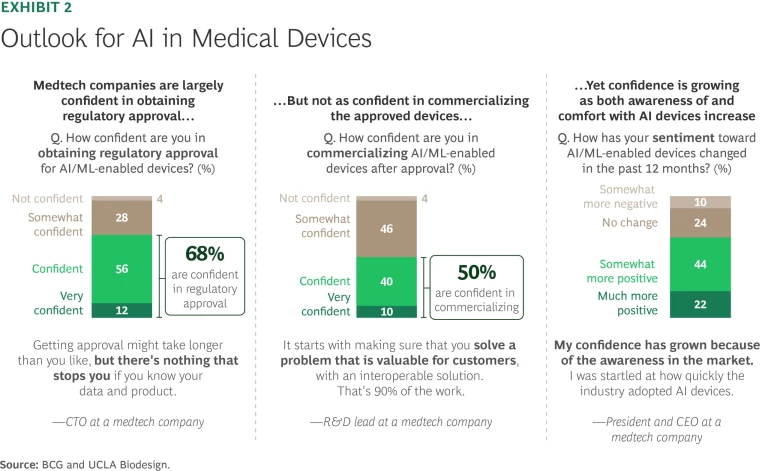
Over the study period from 2010 through Q3 2024, the character of VC investment has evolved. In the early years, most VC funding took the form of preseed and seed rounds, accounting for a staggering 96% of all such investment in 2013. By 2022, however, Series A through D+ funding rounds had become the majority, reflecting the maturation of medtech AI/ML concepts and the more substantial capital required to drive toward product approval and ultimate commercialization.
Payday arrived for at least 58 of the companies tracked in our study. IPO exits were scarce, with just five deals recorded during this timeframe, although both United Imaging (at $1.6 billion) and PrediLife (at $4.0 billion) crossed the $1 billion threshold to attain unicorn status. Of the nine buyouts and leveraged buyouts that occurred during the same period, only one—Esaote Group—fetched over $100 million. Most VC exits took the form of M&A, often by well-established medtech leaders such as Stryker, Boston Scientific, and GE Healthcare.
Meanwhile, a multitude of VC-backed medtech companies await their big payoff. Average postdeal valuations of medtech AI/ML companies stood at around $10 million from 2010 to 2016, but that figure had tripled by 2022. Along the way, investors created at least nine unicorns whose appeal was based, at least in part, on the strength of their medtech AI/ML products. Some of these companies have stumbled in more recent years, however—most spectacularly, the now bankrupt 23&Me.
Whether because of higher interest rates or general jitters about commercialization, deal activity and valuations in the sector have subsided considerably in the past two years. Average deal sizes are now anchored in the range of $15 million to $20 million, with the largest transactions approaching $100 million.
Subscribe to our Health Care Industry E-Alert.
Looking Ahead
The current landscape offers reasons for both excitement and caution. Data privacy and ownership rights continue to challenge the overall environment for AI investments. Major markets outside the US, such as the EU and China, have differing views on how to regulate AI—particularly in areas as sensitive as health care—raising the question of whether FDA-cleared products will have legs in other markets. Regulators must figure out how to approve products that evolve over time and create solutions on the basis of a complicated logic not subject to human oversight.
Still, medtech AI/ML shows tremendous promise in areas such as pattern recognition, risk prediction, signal detection, clinical efficiency, and personalization. New medtech AI/ML products are gaining clearance at ever-increasing rates—a trend that is likely to accelerate if a proposed US Senate bill authorizing automatic Medicare reimbursement for algorithm-based health care services becomes law. That said, the US Department of Health and Human Services and the FDA and are not immune to the sorts of sudden and unpredictable policy changes that other agencies have recently experienced. In the absence of major changes, however, regulators will continue to accrue valuable experience as AI/ML products emerge and become normalized, helping to close the time-to-approval gap with standard products.
Ultimately, widespread adoption of AI/ML-enabled medtech products depends on trust. The medtech companies offering learning devices and the regulators vouching for them serve as stewards of safety and efficacy for the physicians and patients who rely on these products. VC investor Amy Rees Anderson famously pointed out, “Trust takes years to build, seconds to lose, and forever to build back.” As medtech companies stake their claims, company leaders must assemble the right talent and set clear guardrails for patient safety to deliver the wealth of health care innovation that AI/ML products offer.
The authors would like to thank Kalyn Ricciuti (medtech knowledge analyst, BCG, Boston), Matt Sternberg (lead product manager, BCG X, Chicago), Nami Rokhgar (project leader, BCG, Los Angeles), and Jeff Dean (principal, BCG, San Diego) for their analytical work in support of this effort. In addition, we thank Kerri Miller and Miriam Mburu of BCG Global Marketing for their publication support.