Traditional media companies, recognizing that they need to find a path to a brighter future, are just beginning to experiment with robotic process automation (RPA) and artificial intelligence (AI). But few have tapped the full potential of RPA and AI to stabilize their legacy businesses, reduce back-office costs, and create rewarding experiences for their audiences.
RPA and AI are complementary tools—the brawn and brains of performance. In many industries outside of media, companies have improved efficiency through RPA while introducing AI in order to secure a strategic edge. (See “ Powering the Service Economy with RPA and AI ,” BCG article, June 2017.)
Within media , many newer companies, such as Spotify, Netflix, and Facebook, have long embraced both these technologies—and now it’s time for the more traditional media companies to make the same leap. To launch into the world of automation and machine intelligence, media companies can start with low-value, routine activities while liberating humans to focus on creating killer content and other crucial activities. Later, they can harness the cognitive power of machines to bring insight and speed that will enhance the audience experience and improve the creative process.
A Role for Machines in Media
In RPA, software robots take over activities traditionally performed by humans. These bots can open databases and content management systems, copy data between programs, compare entries, and perform other routine tasks. RPA is ideal for repetitive, rules-driven processes that span several IT systems—it’s like a macro on steroids.
The bots have several advantages over humans. They work 24-7, rarely make mistakes, accept new assignments without complaint, are easy to monitor, and operate four to five times faster than humans. Generally, they pay for themselves within a year and help companies increase productivity by up to 80%, depending on the amount and complexity of the manual work they are replacing.
RPA is ideal for repetitive, rules-driven processes that span several IT systems—it’s like a macro on steroids.
For better or worse, RPA is a Band-Aid. It can be laid on top of existing IT systems without time-consuming and costly integration. But software bots are strict rule followers. They neither learn nor improve over time. When rules change or conflict with reality and when unexpected events occur, human intervention is needed.
In addition, RPA decreases the flexibility of the IT architecture. Whenever companies change the underlying systems, they also need to modify the bots that run on top of them. Companies should be disciplined in documenting changes to systems and processes at all times but especially when they are scaling up RPA.
AI, on the other hand, is the realization of intelligent behavior in computers. AI systems process signals and retain knowledge. They can interact deeply and intuitively with the real world and with humans, make decisions in ambiguous situations, and learn from experience. Machine learning (ML) is a branch of AI in which the machines learn with little or no human intervention. In the media industry, for example, companies are using AI and ML to target specific audiences with relevant advertising and content and to automate video selection, annotation, and production. (See “All the Automation That’s Fit for Purpose at the New York Times.”)
ALL THE AUTOMATION THAT’S FIT FOR PURPOSE AT THE NEW YORK TIMES
ALL THE AUTOMATION THAT’S FIT FOR PURPOSE AT THE NEW YORK TIMES
The New York Times, nicknamed the Gray Lady, is getting a makeover but not one that most of its audience will notice. The newspaper has been steadily introducing automation into its operations. While some effects of automation peek into public view, such as the ability to offer reader comments on more articles, most occur behind the scenes. We recently spoke with Nick Rockwell, the newspaper’s chief technology officer, about some of the changes.
What is the current status of automation at the NYT?
We have a strong record of automating our printing operations and are now looking at the digital parts and processes of our business. There are two big differences between our printing operations and digital activities. The first is that our printing operations had been stable for decades, so we were automating fixed processes. Digital workflows are in a constant state of adaptation, so we need to be flexible. The target is always moving. The second is the availability of data in digital workflows. We need the ability to capture, harness, and process this data.
In what areas do you see automation providing the greatest value or benefit?
I think we need to distinguish between customer-facing activities and internal processes. Many of our most valuable customers are reluctant to embrace automation, but in specific areas automation can drive significant value to them. For example, we have applied artificial intelligence to help us moderate our online comments from readers by flagging those that require editorial review. AI has boosted our efficiency by 40% to 50% so that readers can comment on many more online articles.
Internally, we have fully automated editorial and content management workflows as well as the annotation, tagging, and indexing of articles. We have also started to use AI-based automation to suggest photos for specific articles.
How do you drive the adoption of automation within the organization?
We believe in the full independence and freedom of our data science and machine-learning team. This team of highly skilled individuals identifies automation opportunities and drives the adoption in close collaboration with the business units. The team’s independence has helped keep the team engaged and motivated. For example, it recently developed automated digital ad products on its own initiative. We are also willing to hire external expertise when building critical in-house expertise takes too long.
What challenges do you see in introducing automation?
We respect the value that our editorial talent generates. So while we support the editorial team, we are not trying to apply automation to replace them. We also recognize that, while automation helps us to become more efficient, it also leads to uncertainty about what the future will bring. We have dealt with this uncertainty by involving more stakeholders more closely in our projects. We are also spending more time planning and implementing automation-related changes to ensure smooth adoption of the new ways of working.
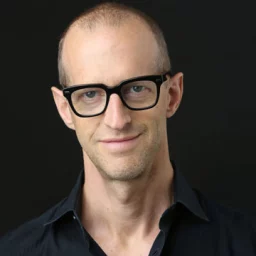
Four Steps to Take Before Introducing Bots and AI
Even companies with legacy technology architectures can roll out automation and AI, and many media companies have started. (See “Media and the Machine.”) Before proceeding, they should take four steps:
Media and the Machine
Media and the Machine
Digital-only media companies such as Facebook, Google, and Netflix have been relying on automation and AI for most if not all of their lives, but traditional media organizations have started to get in on the act.
Xinhua News Agency, China’s state-run press operation, created Magic, a system that can gather, edit, and produce a news video in ten seconds, according to Xinhua. Magic is a composite acronym of machine-generated content and AI.
The Los Angeles Times once covered only about one in ten homicides in its home county, so it focused on the most newsworthy deaths. The paper created an automated method that pulls crime data directly from the Los Angeles coroner’s office and now offers coverage—including a database, interactive map, neighborhood analysis, and blog—of nearly all homicides.
The Associated Press increased its coverage of quarterly earnings by a factor of ten with a platform called Wordsmith that uses natural-language generation to convert data into brief stories. The AP now produces 3,000 quarterly-earnings reports and estimates it has freed up 20% of its editorial staff during earnings season.
Reuters created an AI-enabled tool called News Tracer that scours Twitter to find and verify news accounts. The News Tracer algorithms look for clusters of similar tweets, evaluate their newsworthiness, and then seek corroboration. News Tracer gave Reuters a 15-minute lead on a terrorist bombing in New York City in October 2016.
The Wall Street Journal has developed an AI system that optimizes how much free content to offer visitors before requiring payment, using more than 60 clues, such as operating system, device, and location. Readers who the system determines are likely subscribers hit a paywall much faster than readers who need a nudge to subscribe.
Spotify delivers a weekly individualized playlist of 30 songs that subscribers have never before listened to on the service. Discover Weekly powerfully combines insights generated by three separate engines that analyze behavior (the number of times subscribers listen to a track, for example); news articles, blogs, and other online text about relevant bands and tracks; and the audio files themselves to find similarities among tracks. In 2016, one year after Discover Weekly’s launch, 40% of subscribers had streamed 5 billion tracks, and half saved at least one track per week.
- Clear Vision and KPIs. One effective approach is to set top-down targets that are then broken down by departments. This ensures the strategic alignment of AI and automation projects as well as the development of expertise and capabilities in individual parts of the company.
- Talent and Outsourcing Strategy in Light of AI Scarcity. A key part of the strategy should be a framework for how and when to use external vendors. Vendors play a distinct role in an AI world because they need to train their AI tools using data, which often includes sensitive client information. Media companies need to work with these vendors in ways that strengthen rather than sacrifice competitive advantage while increasing their internal capabilities.
- Identification of Processes to Automate. Companies should identify established processes that can be broken down into discrete tasks. This common-sense requirement is sometimes challenging at organizations whose processes were built in an analog world and rely on the expertise of individual contributors. It can also be tricky when newer digital processes are in flux.
- Data Collection and Strategy. Companies should pull together a sufficient quality and quantity of granular data to feed into the machines and a strategy to acquire data from second and third parties. In building a personalization and recommendation engine for consumers, for example, broad, general demographic data is much less valuable than data about users’ identities, interests, and near-term purchasing intentions. (See “ Media Companies Must Reimagine Their Data for a Digital World,” BCG article, September 2017.)
Three Approaches to Implementing Bots and AI
Media organizations have three options for introducing automation and machine intelligence, depending on their ambition and the extent of their legacy infrastructure:
- RPA Overlay. Rather than replacing old systems, companies can use RPA to extend the life of their existing infrastructure. RPA can automate what is sometimes called “revolving chair processes,” activities that require humans to move back and forth among legacy back-office systems.
- AI-Supported Automation. AI can take over specific repetitive tasks currently completed by humans, delivering results more quickly and with higher quality. It can also perform tasks such as making recommendations on Netflix or Spotify much faster than humans. In this support role, the AI engine generally covers specific self-contained tasks rather than managing an entire process. Even in these contexts, there is typically a human in the loop to monitor performance.
- RPA and AI Combined. As complements, RPA and AI can also work together. Companies can achieve both the rapid payback of RPA and the advanced potential of AI. YouTube, for example, relies on ML to identify potential extremist and terror-related videos. An RPA system then blocks these videos and routes them to a human to confirm that the machine made the right decision. Over time, an AI system can take over more steps in this process, as it starts to mimic human decision making.
For RPA and AI to succeed, the right environment is vital. For RPA, companies need to identify both standardized processes that robots can take over at scale and other processes that are still standardized but less easy to scale because, for example, they may be aimed at specific customer segments.
For RPA and AI to succeed, the right environment is vital.
The degree of difficulty involved in implementing AI is higher than in RPA, requiring a detailed understanding of both the meaning and the quality of the available data. Companies need to identify relevant data and the systems that hold it. They then need to access, collect, and store this data so that it can be fed into decision-making algorithms.
These tasks can be challenging, particularly for media companies such as broadcasters that do not have a direct relationship to their audience. Broadcasters have been working hard to introduce addressable TV, in which targeted ads are inserted into TV programs. But because they do not have access to data on the demographics and interests of their audience, they are at a disadvantage. Farsighted broadcasters, however, rely on AI-based data management to process and triangulate data from connected devices and third parties to build profiles of households and individuals. This creative data collection exercise allows them to target ads to specific audiences.
Many use cases in media organizations are ripe for RPA and AI, including topic monitoring and recommendation, text-to-speech and voice interfaces, dynamic pricing, service activation, and campaign management. (See the exhibit.)
Compared with the computerization of newsrooms several decades ago, the introduction of RPA and AI into media organizations does not need to be prohibitively complex or expensive. The first steps are to identify several promising use cases as pilots, set business goals and KPIs for each pilot, and then establish measures to acquire the data and technological capabilities to achieve those goals.
In moving beyond pilots, the degree of difficulty rises. As companies delve more deeply into automation and intelligence, they discover that the technologies change the nature and economics of work and consequently have significant implications for how companies should organize and deliver their services. AI, for example, is essentially a test-and-learn activity in which machines learn from past results. And the employees working alongside the machines must adopt a similar iterative approach. This way of working may be challenging to the hierarchical setups of newsrooms, broadcast studios, and other media operations.
Vendor management is more critical in AI than in traditional technology areas because these companies have hired so many of the scarce AI professionals. Until more people are trained in AI, the issue remains how—not whether—to work with these vendors. Ideally, media organizations should limit vendors’ access to high-quality, unique data. At the same time, they can buy or license off-the-shelf solutions only in commodity areas such as HR, finance, and customer service optimization, as long as they continually monitor the cybersecurity and compliance strength of the vendors. (See “ The Build-or-Buy Dilemma in AI ,” BCG article, January 2018.)
Until more people are trained in AI, the issue remains how—not whether—to work these vendors.
Ultimately, media companies may be headed toward an “automation and intelligence first” imperative—similar to the “mobile first” or “digital first” mantras of just a few years ago. Media organizations should prepare for this new world by building both understanding and capabilities.
The good news is that machines can provide step-change improvements in efficiency, performance, and insights. In the process, they will free humans to do what they do best, which is to tell compelling stories, create engaging customer experiences, and make connections with their audiences.