With so many moving parts and interdependencies, a lot can go wrong with transformation initiatives. Often, snags arise and spread long before transformation leaders are even able to notice. And when things go south, that can put the whole transformation in jeopardy.
Transformation is always a high-stakes undertaking, but in times of heightened external uncertainty , setbacks can have greater consequences. Organizations need to reduce the uncertainty of these high-stakes efforts early on to boost the odds of success. But how?
By unlocking and synthesizing insights and lessons—theirs and others’—through a groundbreaking approach that combines the power of organizational data with analytics , AI, and machine learning.
The Challenges of Predicting Initiative Success
A typical transformation at a large organization can take hundreds, if not thousands, of initiatives to carry out. These initiatives cut a wide swath throughout the business. Measuring initiative progress has typically been laborious; with all the metrics to input, timely analysis is hard to accomplish. This analysis is also subjective because it is survey based and expertise dependent. And because analysis can only happen retrospectively, companies aren’t easily able to head off a derailment or failure before it becomes irreversible. In fact, an initiative might appear to be on track until the last moment, when it isn’t.
AI and machine learning change all that. A data-driven approach BCG developed eliminates the inevitable bias that comes with traditional approaches. It allows for comparison not only against a company’s own prior transformations, but also against large data sets from the transformations of other companies. In addition to boosting statistical significance, this approach establishes benchmarks that can help inform risk mitigation and decision making, whether at the initiative level, organizational level, or for the overall transformation.
In effect, this approach formalizes the experience factor, making understanding and insight gathering an institutional capability. By using leading and lagging indicators, AI enables companies to adjust initiatives and targets based on real-time information. These real-time information flows and forward-looking capability contribute to a company’s overall ability to sense and adapt to external change—in other words, they help build resilience .
AI enables companies to adjust initiatives and targets based on real-time information.
Once set up, leaders can run the analysis as frequently as they want, including continuously, and at low cost using their own data. The expertise is delivered to the right people to shape the model. Companies can thus monitor initiative progress in real time and predict slippage and problems so they can keep the initiative on track. The outputs also provide leaders a clearer picture for risk mitigation: a single repository that supports the transformation management governors, such as executive steering committee, transformation management office, and regional or divisional TMOs. The consolidated data, tracking and reporting, monitoring, and predicting give these teams what they need to ensure financial discipline and accountability and the visibility to course correct.
A Real-Time AI Approach
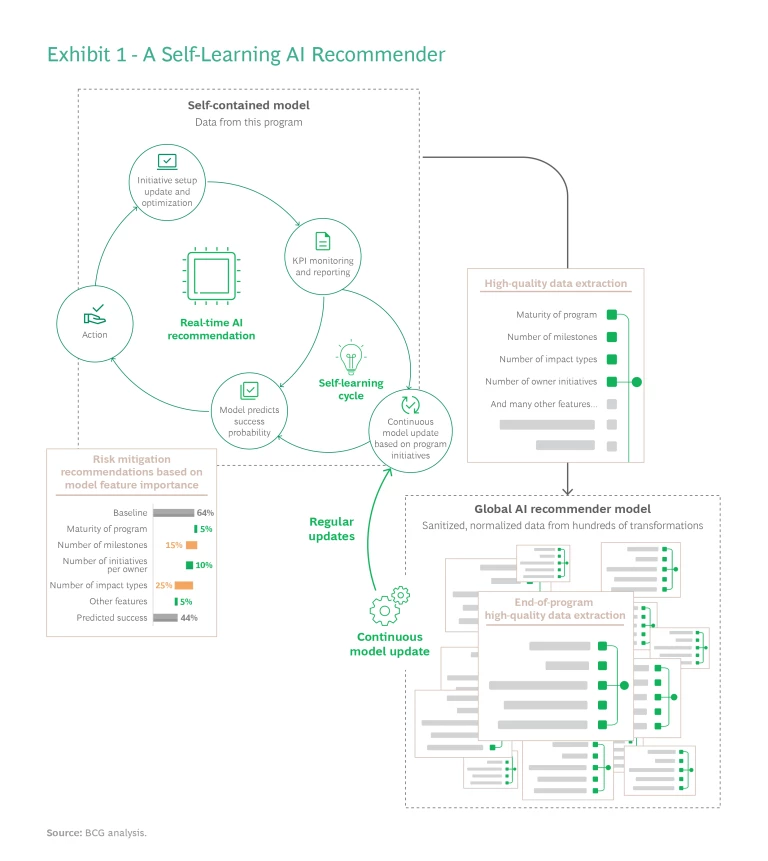
Using a rich data set of tens of thousands of past transformation initiatives, we developed a machine learning model that considers the individual context of initiatives to predict the probability of success on a holistic level. This method—the self-learning, data-driven AI model—predicts initiative outcomes in real time and helps to navigate complexity and codify best practices.
From these findings, we came up with the concept of an AI “recommender,” which runs in real time and alerts initiative owners, TMOs, program management offices (PMOs), and program managers of initiative status, current projections, and recommended actions. (See Exhibit 1.) And while this recommender is integrated into KEY , BCG’s program management software, it is not a black-box approach. Its methodology is applicable to any transformation program.
To begin, the company enters the initiatives into the program management software, along with performance KPIs and the lagging indicators that measure current production. Once set up, new data will be continuously fed into the software in an ongoing cycle. Next, the PMO and team members perform a quality check to be sure initiatives have been carefully designed and are as bulletproof as possible. Such a check can be formalized using a rigor test (a discussion among key stakeholders for testing initiative quality) and a “health” report that dissects the factors driving success throughout the initiative’s life span. (See Exhibit 2 for a simplified example of a health report.) In a separate process, PMO members verify that all necessary data is provided and approved by finance , HR, the PMO, and other parties.
The recommender uses a model to predict in real time a success rate that you can measure and explain. We seeded this model by anonymizing, importing, normalizing, and learning from 64,000 past initiatives from a large set of company transformations. This seed model is not essential, but it dramatically enhances the starting model right from the start. Based on the weighting of your results, the model offers a risk mitigation recommendation. From there, you can run simulations, testing the effect of tweaking certain characteristics, like “what if we cut the number of milestones by a third?” Or “what if we held off initiative x until initiative y was finished?”
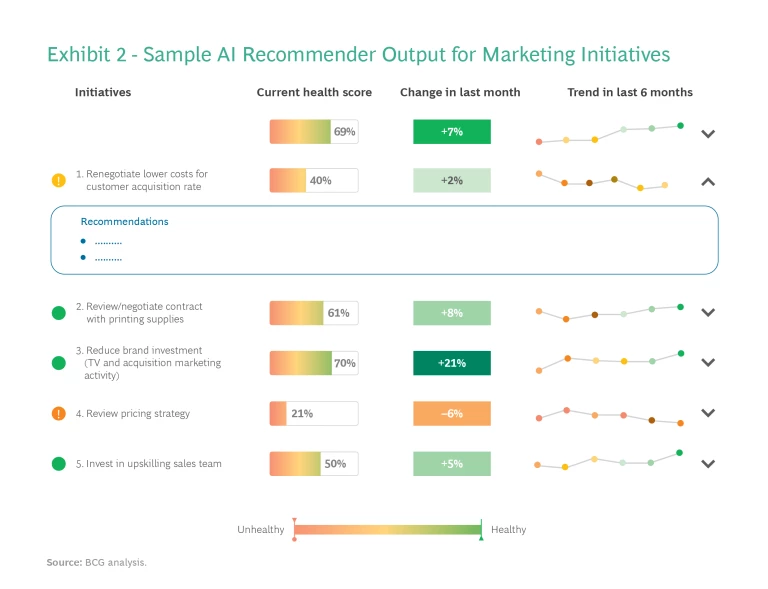
The adjacent “self-learning” cycle is the heart of the data-driven approach. Here, the model is continuously updated, with new data and new patterns extracted that show, for example, baseline versus best practice results according to different variables, including program maturity, number of milestones, number of impact types, and owner experience. With new data fed into it, and the company’s own addition of data, the algorithms improve over time.
The recommender function learns by comparing its predictions with the actual results and adjusting accordingly. It can alter the weighting of specific criteria. Some companies, for example, put particular emphasis on financial impact metrics, in which case the model would weight such metrics accordingly. The secondary learning cycle is enabled by the analysis of the anonymized transformation data of other companies.
This means, for instance, that companies can adjust targets more realistically. Suppose leaders set a target of cutting paper procurement by $10 million a year, but that in that first year, despite best efforts, procurement cost cuts only amounted to $6 million. With the company’s own historical data on similar efforts, augmented by data from thousands of other similar initiatives, the company can adjust the target confidently, without guessing. This ability is especially helpful with multiyear initiatives; after the first year or two, there is less waste to identify to eliminate, but without solid data to go on, it can be difficult to know how much to modify a target downward without overdoing it.
A company can still use ancillary tools to complement an AI model. We did not conceive of the AI tool we use as a replacement for BCG’s DICE indicator, a survey-based tool that relies on objective measurements used to calculate a success-likelihood score. In fact, using both together will heighten the predictive and explanatory
The self-learning, data-driven AI model predicts initiative outcomes in real time and helps to navigate complexity and codify best practices.
Four Rules for Initiative Success
A paradox of transformation is that the first initiatives undertaken, which are typically the top-priority initiatives, tend to have a higher failure rate. A data-driven approach can help leaders jump-start their learning, leveraging their own data with the help of algorithms and continuously generating new insights that enable them to fine-tune their approach over time.
Insights can also guide help mitigate against the inevitable imperfections that every initiative effort sustains. No organization can address all the flaws at once; but insights help illuminate the tradeoffs and guide choices, gauge the impacts, and course correct. When the algorithm senses an opportunity to improve on initiatives, adjustments can be made more quickly.
An important benefit of these algorithm-generated insights is that with so many more ways of observing patterns and connections, indicators that once served on a standalone basis can now support a holistic perspective. For example, the question of too many milestones may or may not be an issue; but when it can be linked with other characteristics—say, initiative rhythm or the expertise of the initiative team—it has more meaning. Importantly, algorithms are not meant to replace the human interactions between the transformation management office (TMO) and initiative leaders. Rather, they facilitate and enrich those interactions, thus boosting not just the odds of success but its degree.
There is no ideal setup for an initiative to be successful, but from our analysis of thousands of real-world initiatives, we distilled four golden rules for initiative success. When applied together, they all reinforce each other.
From BCG’s analysis of thousands of real-world initiatives, we distilled four golden rules for initiative success.
Strive for Simplicity. Our analysis shows that complex initiatives tend to fail more often than simpler ones. Some are complex by being overly overambitious. For example, they may have too many KPIs or too many milestones—30 where 10 would suffice. Ambiguity is sometimes the culprit: “increase in procurement efficiency” can easily be interpreted differently by different people.
It’s important to break complex initiatives into more manageable pieces. Train owners how to define and manage milestones and simplify their scope. Actively monitor against complexity “creep” across the entire initiative portfolio. An AI model can help continuously monitor for complexity traps, using data points and financial and time metrics to set off alarm bells. Some of these elements can also be addressed by keeping the program parameters—such as the number of attributes and the complexity and variance of success KPIs—simple and limited from the start.
Set a Brisk Rhythm. By the four-month mark, you usually have an idea whether an initiative is destined to succeed or fail. The biggest clue? Rhythm: how quickly it must hit milestones and the pace of transformation office activities such as meetings with initiative owners or frequency of stage-gate reviews. Initiatives that follow a brisk pace, with shorter intervals between milestone deadlines, generally have a 20-percentage-point better chance of delivering their full potential.
Subscribe to our Artificial Intelligence E-Alert.
Furthermore, initiatives launched later in the transformation tend to perform better, largely thanks to the learning effect. Over time, teams get better at forecasting impacts once the low-hanging fruit is harvested and associated distortions are eliminated. So, establish an energetic pace, limit the time between milestones (especially in the beginning), document lessons, and actively disseminate them.
Flag Issues Early On. Often, even make-or-break initiatives can veer off track. If the slippage goes unnoticed by transformation leaders, teams may alter targets to avoid red flags in the management reports. This not only leads to significantly lower realization rates, but it also masks execution problems that could have been addressed or averted.
For these reasons, exception-based reporting (whether done manually or automatically) and forward-looking reporting are vital. Issues that are corrected with transformation leaders involvement tend to fare better than those that initiative owners manage themselves. The TMO has the purview and the power to address shortfalls, help mobilize additional resources, and prioritize when interdependent projects are competing for the same limited resources. It can also alert senior management about the impact of delay on interdependent initiatives.
Tap the Experienced. Every transformation contains key initiatives that are the building blocks of renewal. Managing any one of these pivotal projects is highly specialized, intensive work that calls for a seasoned, top performer. Such initiative owners should have a deep understanding of what it takes to set an initiative up for success and direct experience in leading complex, time- and impact-critical initiatives.
Having the right standing within the organization is also important. Initiatives governed by those responsible for multiple initiatives perform better. Compared to owners of one or two initiatives, multiple-initiative owners have about a 25-percentage point greater likelihood of success. Companies need to appoint the right people in the right places and treat experience and responsibility as key success drivers. The more initiative leaders learn over time and the more they grow into their role, the more responsibility they can take on.
Until recently, the best companies could do to improve initiative outcomes was conduct retrospective analysis. Given the volume and complexity of transformation initiatives and their interdependencies, correcting course was tough to do.
Big data and the power of AI now make the once impossible a reality. By harnessing the power of initiative data, companies can now have a window into actual performance as it unfolds. They can capture insights once unobtainable, synthesize them with a stockpile of timeless insights, and apply both to boost the success of current and future transformation initiatives.