We are in the midst of a global data and analytics revolution that presents huge opportunities and benefits for Australian companies and governments; yet Australia is an AI laggard. Australian businesses and government agencies have been slow to adopt AI and drive value from investments. What will it take to accelerate the process of turning the exciting potential of AI into a safe, value-creating reality?
The Promise of AI
AI (defined in this article as the broad use of advanced analytics, including machine learning) is one of the main forces that will transform industries over the next decade. According to Stanford’s latest AI Index, US$93.5 billion was invested globally in AI in 2022, and a BCG-MIT survey revealed that 84% firms believe that AI helps them to gain a competitive advantage.
AI has the potential to transform performance in four ways (illustrated by Australian examples):
- Optimization – using analytics to optimize an outcome (such as cashflow, yield or on-time departure) for a given set of decision variables, objectives and constraints. For example, Woolworths use optimization algorithms to localize products stocked in each store and to calibrate timing and discount depth of promotions.
- Personalization – using customer insights to deliver the right message to the right customer at the right time in a way which is valuable for customers and delivers business outcomes such as reduced churn and increased share of wallet. For example, a number of Australian retailers are already using machine learning to personalize loyalty offers and product recommendations through email and app channels.
- Automation – involving the digitization of labor-intensive activities. In industries as diverse as insurance, healthcare and manufacturing, use cases such as same-day claims payment, more accurate interpretation of medical images, and use of facial recognition and biometric verification are delivering large productivity and customer experience benefits. For example, IP Australia uses AI to support text and, increasingly, image matching for trademark applications in the pre-application process, saving Australian businesses time to see if their application will likely be successful.
- Predictive operations and maintenance – involving the use of data (such as manufacturing, operations and supply chain data) to monitor, simulate, predict and evaluate processes. For example, Endeavour Energy uses AI-enabled computer vision to review and assess real-time footage of assets, assess for damage and prevent faults before they occur.
The Benefits of Well-Deployed AI Are Transformational, Not Incremental
The financial benefits of AI are proven, even if only a small percentage of companies have realised them to date. For example, optimisation often reduces in-scope operating costs by 15–30%, and personalisation can increase revenue by 6–10%.
There are many more advantages beyond purely financial outcomes. Companies that have successfully implemented AI solutions report major improvements in culture and collaboration. 78% of teams that had implemented AI reported better collaboration vs teams that had not implemented AI.
Further, as customers use digital channels more and more to research, transact and manage their purchases, AI can be a real differentiator by tailoring the customer experience. For example, in the Australian retail context, digital advertising has grown to a $10 billion+ industry. E-commerce adoption increased from 10% to 15% across all industries during the COVID-19 pandemic and is expected to increase further.
Governments also have a policy imperative to provide personalised services to citizens. BCG research shows that over 90% of citizens are comfortable with governments using citizen data to deliver more personalised services.
In summary, bluntly, adopting AI is not a choice – it’s an imperative. The rewards will accrue to the organizations that adopt AI faster and better than their competitors.
There Is Some Good News: The Australian Landscape of AI Providers Is Evolving Rapidly
Australia has a strong local presence of ‘hyperscaler’ infrastructure providers (Microsoft Azure, Amazon Web Services and Google Cloud Platform). Government-backed initiatives are also supporting the build and deployment of local AI capabilities for the benefit of the wider Australian economy. For example, CSIRO’s Data61 is driving AI innovation in health, natural resources and the environment, aging and disability, and cities, towns and infrastructure.
A myriad of local AI-focused application providers target specific niches and use cases, such as:
- Pitcrew.ai and AMLAB are pioneering the use of computer vision for asset management in heavy industry to improve operational efficiency and safety
- Hillridge Technologies has built a weather index insurance platform that better connects to underwriters, reducing financial risk for farmers
- Orefox and SensOre are using AI to predict better targets for mining exploration and improve the effectiveness of drilling investments
- Airwallex is using predictive capabilities to detect suspicious behaviors and proactively prevent account takeovers, reducing the risk of cybercrime to credit card users.
Despite the Promise, Adoption Is Slow and Australia Is Lagging
Adopting and delivering AI is hard. BCG-MIT’s research reveals that only 11% of companies globally report significant delivered cost or revenue benefits from investing in AI.
- Implementation challenges: Companies often do not have the capabilities, enablers, talent, culture, governance and processes to translate good AI ideas into realized value. They are often clear about ‘what’ will drive value, but fail by relying on traditional skills and approaches for ‘how’ to implement. While we see some successful AI solutions at a product level or for a specific use case, embedding AI more broadly is something that few organizations have achieved.
- Risk aversion to data sensitivities: Leaders are often reluctant to drive value from data where there are concerns about whether customers trust companies to use their data, or whether there are real or perceived risks to brands and reputation.
Australia is lagging global peers in addressing these barriers. Around 70% of Australian organizations have yet to succeed in delivering digital transformation
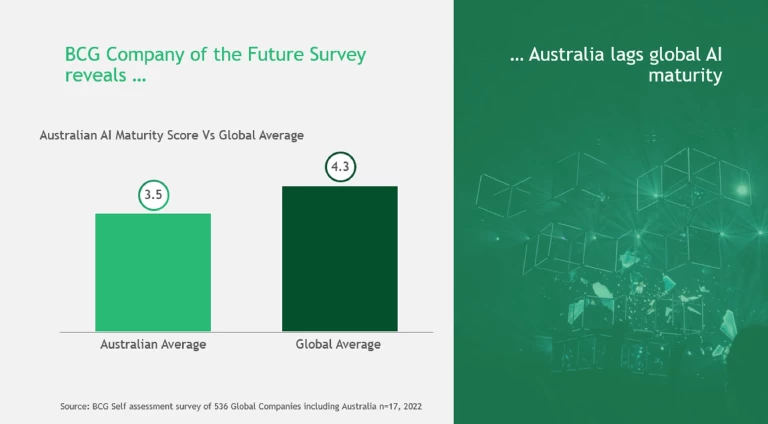
Furthermore, Australia lags other nations on measures of AI investment and innovation:
- Australia invests only one-sixth of the amount that Israel invests in AI, and one-third of the amount that Singapore and the US invest (based on the ratio of private investment in AI to GDP
. ) - Australia is ten times less innovative than Israel, five times less than Singapore and three times less than the US (based on the number of AI companies founded between 2013–2021 as a ratio to GDP
. ).
Why is Australia lagging? There are several reasons. We are underinvesting in AI compared to leading countries. We have less mature privacy regulations, for example compared to European companies and GDPR (General Data Protection Regulations). Those interviewed point to excessive risk aversion at board level around data usage. And recent large-scale local cyber-attacks have put security and privacy risks firmly at the front of mind of business leaders.
How to Overcome the First Barrier: Successful Implementation and Scaling
Six success factors underpin a successful programmatic implementation of AI:
- An AI strategy that links the organization's overall purpose and strategy to specific AI initiatives and business outcomes
- Leadership commitment and perseverance to build AI capabilities
- Access to high-calibre talent
- Agile governance to drive effective cross-functional ways of working
- Monitoring progress towards defined outcomes
- Building a business-led modular technology and data platform.
Only about 30% of Australian companies have addressed all these factors sufficiently, and an insufficient or ‘best efforts’ approach dramatically increases the risks of failure. We explain these success factors below, along with insights into how Australian companies are addressing them successfully.
Success factor 1: An AI strategy that links the organization's overall purpose and strategy to specific AI initiatives and business outcomes
Companies need a clear line of sight between their purpose and strategy, and their AI aspirations. This will translate into specific AI initiatives that drive business outcomes required by accountable business executives. Too often, AI initiatives are peripheral, and owned by passionate technologists but largely ignored (and often resisted) by the business. This leads to many proofs of concept (PoCs) but not to broad adoption. A common failure mode is ‘letting 1000 flowers bloom’ with a corresponding lack of focus and dilution of scarce talent and funding.
Qantas Loyalty Executive Manager for Technology, Data & Analytics, David Rohan, emphasized: “When we’re starting on an AI project, we bring our corresponding business stakeholders along on the journey.” Kim Krogh Andersen, Group Executive of Product and Technology at Telstra, said that “for true AI adoption, AI must be embedded in every mission, just like we do with software, integrated in a holistic manner” rather than being a discretionary afterthought.
An organization's AI strategy needs to be distilled into discrete business use cases with clear problem statements and corresponding measures of value and risk. David Rohan at Qantas warned that if you “throw AI at a problem you don’t understand, the risk of getting it wrong is much higher,” but this can be mitigated by “having absolute clarity on the value you are driving for the customer.” He re-iterated this with a simple acid test: “The first thing I ask is: What are we using the data for? What is the value we are aiming for?”
Woolworth’s Chief Analytics Officer Amitabh Mall shared that without “a clear enough strategy,” an organization is at risk of “too many unrelated pet projects,” and the risk of technologist-driven PoCs rather than business-owned products becomes a reality.
The pass/fail test for this success factor is: Do the business owners need successfully deployed AI solutions to meet their business goals? Are the business owners themselves visible drivers of adoption?
Success factor 2: Leadership commitment and perseverance to build AI capabilities
Executive commitment and top-down conviction to build AI capabilities over a multi-year period is essential. Building any new capability is typically harder than incrementally improving existing ones, and this is true for AI.
Leaders need to acknowledge this multi-year commitment and show perseverance beyond in-year initiatives with support for sufficient and persistent investment and people resources for AI initiatives. Further, they need to engage and motivate middle managers, who may be concerned about job security, uncomfortable with new or unfamiliar capabilities, and cynical about the organisation’s ability to adopt AI. As one Australian executive reflected, “I wonder what percentage of executives in my company really understand and believe that this is not a choice but an imperative?”
Marat Bliev, General Manager, Data, Analytics & Insights at Endeavour Energy, agreed: “We have support from the CEO down to middle management. It’s impossible to get these transformations over the line without support from the top.”
According to Qantas’ David Rohan, the agile approach of starting with smaller use cases to prove value is key to driving understanding and commitment from middle management: “If users have been taken on the journey of using data to solve their problems, this helps them accept the ‘black box’,” and become more comfortable with AI becoming integral to their organisation’s success.
The pass/fail test for this success factor is: Do your executives, and the relevant middle managers, have genuine conviction about the strategic imperative of AI?
Success factor 3: Access to high-calibre talent
Finding, retaining and developing data and analytics talent is hard. Talent pools in Australia are thin, and many data specialists don’t consider working for traditional companies that appear not to prioritise data as a strategic asset. As a result, companies need a highly attractive and credible Employee Value Proposition (EVP) for this hotly contested talent pool. As Telstra’s Kim Krogh Andersen shared, “AI people want to be involved in solving the world’s biggest problems.” Woolworth’s Amitabh Mall agreed: “It is not enough to attract good talent. You need to have a genuine growth mindset and have people work on exciting tasks where they can use their analytical skills to achieve important outcomes, otherwise the best will leave.”
Delivering a strong EVP requires authentic, persistent commitment and creative thinking. “We have created a strong sense of purpose, linking this to our AI aspirations…but we still have issues finding the right talent,” said Michael Schwager, Director General of IP Australia. “If you don’t have the lure of Silicon Valley unicorns, how do you win the best people?” Schwager shared two things that have helped him build high-calibre AI talent: firstly, training their large STEM workforce to be credible data analysts; and secondly, embracing radical flexibility in work location.
Bliev from Endeavour Energy shared that they “picked the best” when scaling new data teams as they wanted to make Endeavour a motivating, high-energy place where people would come and stay. “This is one of the things we have done well, and there are very few leavers.” And the final proof: employee engagement for Endeavour Energy’s data practitioners is 30% higher than for the general workforce.
The pass/fail test for this success factor is: Have you created an effective AI talent plan, taking into account your relative ability to attract, retain and motivate data and analytical talent?
Success factor 4: Agile governance to drive effective cross-functional ways of working
How leaders govern AI initiatives and capability builds is critical. Firstly, the governance must be flexible and adaptive, unlike, for example, traditional cost-cutting programs. Senior leaders need to visibly support AI initiatives and take the time to find ways to unblock issues when they are escalated.
Secondly, leaders need to view setbacks as opportunities to learn and improve communication and decision-making. Bliev at Endeavour Energy stressed, “I encourage rapid escalation of issues which are blocking progress…my team feels comfortable calling me at 10pm at night,” so he can discuss, resolve or further escalate the issues. He added that his leadership team is engaged in making decisions to progress the key AI initiatives on almost a daily basis – a very different cadence from monthly steering committee meetings.
Thirdly, leaders need to drive mission-oriented, cross-functional ways of working, and embed data and analytics experts in teams with all the necessary skills to deliver the business outcome. Qantas’ David Rohan described this as “centralised for governance, consistency and availability, but decentralised for impact.” He implements this with business co-sponsorship of use cases and embedded data practitioners in the business. This cross-functional way of working keeps his data and analytics team “connected to customer value.”
Finally, executives need to align their views on the critical path to deliver AI solutions. One Australian executive said, “There is often a vast difference of views on the enablers – for example, clean and accessible data, effective tools and platforms for testing and then scaling AI deployments – required for effective deployment of AI solutions. So we have different expectations around how much time and effort is required to put these in place.” Agile governance drives alignment of views on time and effort so that executives can actively work together to support progress.
The pass/fail test for this success factor is: Have you created a governance structure in which teams can share their challenges honestly? Do you then actively support them to deliver the best outcomes at speed?
Success factor 5: Monitoring progress towards defined outcomes
Clarity about how inputs (e.g., design, experiments, training algorithms) drive both financial and better risk outcomes is critical. Clarity refers to more than just producing results around, for example, A/B testing of multiple outbound marketing campaigns. It is about developing transparent ways to measure the progress of initiatives (e.g., the first phase of personalisation), capabilities (e.g., clean and accessible data), outcomes (e.g., better customer retention or lower fraud losses) and monitoring of ongoing risks (e.g., what customer data to retain or purge).
“Analytics projects have the ability to ‘wander’ and we need to constantly challenge ourselves to see if they are really delivering on the original intent,” said Michael Gatt, Chief Operating Officer at AEMO. “And robust benefits tracking is critical. You must be able to relate the money you’ve spent to the benefits delivered,” Gatt added.
Endeavour Energy’s Bliev re-iterated this: “In most of the cases, the ultimate metric of success for our use cases would not be the rate of usage of the tool but the value outcome we are aiming for.”
The pass/fail test for this success factor is: Do you have robust, trusted ways to measure investments, delivered value, risk and RoI around initiatives? Do you have ways to measure relevant improvements in capabilities and enablers?
Success factor 6: Building a business-led modular technology and data platform
A technology and data platform that is modular, secure and designed based on business needs is critical for successful AI projects. For example, having a decoupled architecture enables data from different repositories to be easily combined to support a business outcome. Significant investment is often needed to modernise legacy platforms to meet these criteria. A business-led approach allows for the right trade-offs to be made between current and potential business value and the specifications of the platforms required to deliver this value. All too often, technologists design architectures with specifications that are uncoupled from business needs, leading to unnecessary capital spend and delays.
“We are now taking a more strategic view of data, systems architecture, and of vendor offerings,” said Michael Gatt at AEMO. “The days of vendors offering an all-singing-all-dancing platform to suit all possible needs are over…there is no single vendor solution that does this.” He adds, “The entire policy horizon will change by 2025, so anything we are building now needs to be future-proofed, but we don’t know those requirements now. And the technology offerings will also evolve. So we have to design for immediate needs and for future flexibility.”
A modern architecture is also secure by design. In light of the evolving cyber threat landscape in Australia, according to Kim Krogh Andersen from Telstra, “It’s very seldom that new stuff gets hacked; it’s usually the legacy stuff. It’s very important we use new technology to protect us.”
The pass/fail test for this success factor is: Does your technology architecture and data model enable effective access to required data, and for teams to experiment and innovate without driving large integration workloads? And are you confident that you have invested sufficiently in technology, while being business-led to prevent over-investment?
How to Embed AI in Your Operating Model
How to embed AI in your operating model
- Start with a ‘discovery’ stage to describe and quantify the benefits of AI
- Launch focused pilots to demonstrate tangible ‘point solution’ successes. An example might be reducing customer churn in one segment or product, or improving manufacturing yields on one production line
- Invest simultaneously (and iteratively) - in the technology and data foundations to support the portfolio of use cases
- Grow beyond point solutions with broader initiatives and use cases that embed AI across the organisation
- Increase investment in talent, data capabilities and technology to scale these capabilities can be scaled across both production and consumption
- Establish effective governance to assign resources to the highest value areas and ensure that impacts are measured
How to Overcome the Second Barrier: Manage Risks and Build Customer Trust with Responsible AI
Many Australian boards and executives are wary of being too ambitious with AI, for fear of being perceived as misusing customers’ data and losing their trust. They are rightly concerned with two questions:
- How do we secure and prevent customer data from unauthorised access?
- What are the appropriate ways to use customer data to deliver value to all parties?
Answering these questions and adopting AI effectively calls for Responsible AI (RAI) – the ethical, fair, and transparent development and use of AI – with transparency, data stewardship, and leadership and direction that cascades throughout the organisation.
“A simple trust litmus test”
Australian customers have made their concerns about data misuse clear
Recent BCG research showed that 74% of Australians are willing to consent to use of their personal data if the value exchanged is fair
More Sophisticated Data Stewardship Is Critical for Rai
The reality is that organizations need to be more sophisticated about both the value and the risks of data and AI. Good data stewardship has many elements: Compliance – with the narrow but important legal and regulatory requirements; Customer lens – customers want to be informed (in clear language) about how companies gather and safeguard data about them, and they want to understand the different ways in which companies use personal data; Intent vs reality – companies often do a great deal to document how they handle data. However, compliance is harder to guarantee; Technology security – Boards and customers often don’t understand the details around issues such as on-premises, public cloud and private cloud storage, and different authentication technologies and device security. Organisations need a clear view of real and perceived technical risks, and how to address and communicate them.
Four Steps for Building Trust in AI – Internally and with Customers
Best practice organisations design and adopt a specific approach to RAI that starts at the top and extends to operations and product development. There are four steps to building a robust RAI program:
- Empower responsible-AI leadership a good starting point is to appoint a leader to design and run the program, as RAI needs to start at the highest level of an organisation
. - Develop principles, policies, training and communications AI leaders need to extend and cascade RAI to operators and developers, including embedding RAI into processes. For example, Macquarie Bank’s Ashwin Sinha states that they “Avoid training models with PII (Personally Identifiable Information) data,” given that “the vast majority of machine learning models can be trained without PII data”
- Establish robust governance that is integrated into existing risk processes. One Public Sector Chief Data stated that “The required legal and regulatory frameworks are not there yet to manage AI effectively, and hence RAI is a crucial tool to develop confidence internally and trust with end users”
- Conduct structured reviews of AI systems with robust technology risk assessments. These assessments will need to balance the uses of data with customer and user expectations, to ensure ongoing RAI while still creating value for the company. These results can inform ongoing RAI governance and continuous improvement.
Today only 21% of companies around the world feel that they have a fully mature RAI program in place, and most Australian companies have work to do to feel confident about both managing the risks around data as well as extracting the value from it.
The Way Forward for Australian Companies to Drive Value from AI
Building a set of AI capabilities that delivers competitive advantage is a strategic imperative. As Telstra’s Krogh Andersen said, “There will be two types of companies in the future: those who see the threats around data and revert back to conservative, tried-and-trusted data stewardship; and those who will seek to understand the power of data, and build new capabilities to add value as well as to manage the risks. The second type of company will win.”
Australian companies that continue to lag will rapidly become disadvantaged and fail to realise the transformational value from optimisation, personalisation, automation and predictive operations. To unlock this value, leaders need to address the two barriers to AI adoption: overcome implementation challenges by addressing the six key success factors; and overcome risk aversion to data sensitivities by building a Responsible AI approach.
The data available to use in AI is doubling every 18 months or so, which means that both the opportunities and the threats are escalating exponentially. The impact of the arrival of Generative AI has served as a wake-up call. Catching up, and getting ahead, is an urgent priority for Australian companies, government agencies – and for the country as a whole.
Postscript: We would like to acknowledge the contribution of the late Marat Bliev to this work and his insight into the value of AI. With condolences to his family, friends and colleagues.