In just a decade, artificial intelligence (AI) has transformed from a promising research topic into an accessible and pivotal technology at the center of a new industrial revolution. AI is reshaping fundamental processes and functions across entire industries, from drug development and airline scheduling to supply chain optimization and medical imaging. AI is no longer a concept of the future—it’s a game-changer today. And companies that move ahead decisively and strategically with AI will gain significant lasting advantages within their industries.
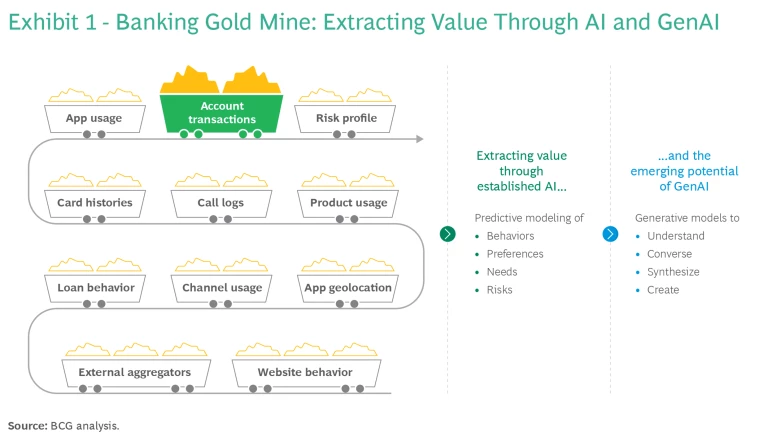
Nowhere is this more evident than in the financial sector. AI runs on data, and banks and other multiline financial institutions (FIs) command vast, high-quality, customer-centric gold mines of data. In particular, the granular transactional data of a bank’s customer base can provide precise, wide-ranging insights into behaviors, preferences, needs, and risks in ways that few other industries’ data sets can. (See Exhibit 1.) For instance, in retail banking , leveraging AI to forecast and tailor future product offerings on the basis of customer needs and behaviors is rapidly becoming table stakes in many banking markets.
The rise of generative AI (GenAI) has enriched the broader AI toolkit, accelerating opportunities for financial institutions to create new value with AI. The ability of GenAI models to digest (understand) and generate (converse) in plain language makes AI capabilities more universally accessible, extending the reach of AI assets to nontechnical users throughout organizations.
FI executives should take the arrival of this new phase of technology as an opportunity to commit to AI and GenAI as key drivers of the industry’s future direction.
In this article, we chart a roadmap for this journey, from integrating GenAI into existing frameworks to reimagining traditional
operations
through a complete AI transformation. In the rapidly changing AI landscape, establishing a firm people strategy is as critical as adeptly navigating the challenges of governance and regulation. And because the technology progresses daily, a forward-looking AI vision is imperative for financial leaders shaping the future.
Incorporate GenAI in Your Roadmap
Media headlines tend to cast an exaggerated and often imprecise spotlight on GenAI. The resulting hype and confusion have caused many executives to question whether GenAI will render their existing AI strategies and initiatives obsolete. The clear answer is no. Indeed, to the contrary, GenAI complements AI that is already embedded in existing FI strategies.
Many people in the financial sector informally use the term AI to refer to a subset of AI techniques that focus on predictive decision-making models. (See Exhibit 2.) Over the past decade, this form of AI has risen to prominence in FIs primarily because it addresses various prediction and classification challenges that are pivotal to banking and insurance , such as risk monitoring, optimal pricing, and product propensity modeling. We refer to this form of AI as predictive AI.
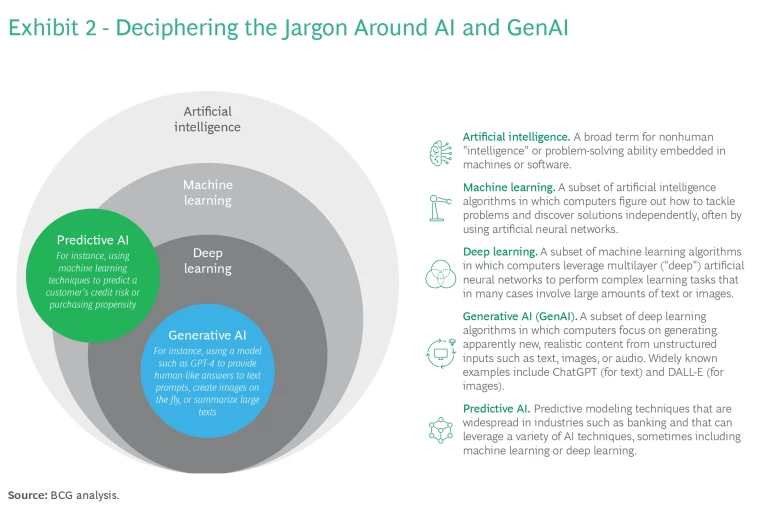
GenAI and predictive AI are powerful tools, but they serve fundamentally different purposes. Consequently, using them is not an either-or question. A bank’s AI strategy will need to include both of them going forward, harnessing their respective strengths in different ways.
One way to think of how predictive and generative AI complement each other is on the model of the two halves of the human brain. (See Exhibit 3.) Predictive AI is comparable to the left side of the brain, wired specifically for logic, measurement, and calculation. This left brain comprises algorithms that assign probabilities, categorize outcomes, and support decisions. For its part, GenAI acts as the right brain, wired to excel at creativity, expression, and a holistic perspective—the sorts of skills required to generate plausibly human-sounding responses in an automated chat.
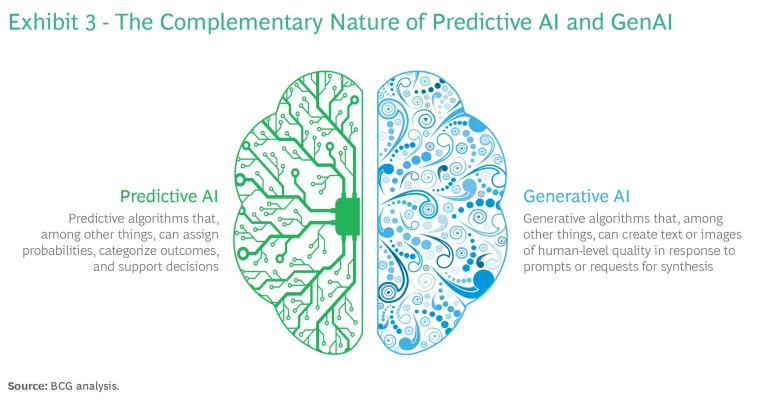
Rather than negating the fundamentals of existing AI strategies, GenAI adds a new skill set to the mix. Accordingly, leaders should lean in and consider how GenAI can enhance and extend their current AI approaches by opening up new opportunities for AI-driven impact.
Many people may initially associate GenAI in banking and insurance with customer service chatbots, but the technology’s versatility extends far beyond these applications to encompass tasks such as automated financial analysis and AI-assisted code development. Numerous global banks are exploring such uses for GenAI models (either built in-house or sourced as a service), and industry giants such as Goldman Sachs, Deutsche Bank, American Express, and Wells Fargo are already starting to go live with their solutions.
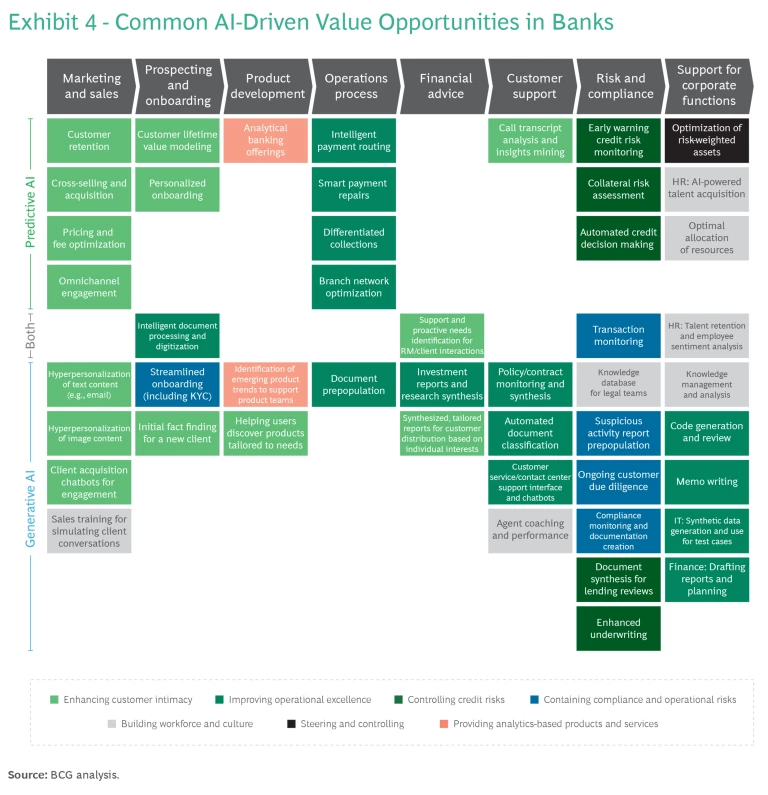
When considering the new opportunities of GenAI alongside existing predictive AI–driven solutions, leaders should bear in mind that well-proven and potential AI applications now span almost every aspect of FI workflows, from client-facing roles to back-end operations. (See Exhibit 4.)
To take full advantage of these new GenAI opportunities, financial institutions must sharpen their methods for identifying, prioritizing, and incubating initiatives that are likely to have the greatest positive impact on value generation, customers and employees, and quality. Two guiding principles emerge for leaders: be clear about AI’s strengths and weaknesses, and take a disciplined approach to AI experimentation.
The Boundaries of AI’s Capabilities
As with any tool, it’s important to use AI in suitable applications. In the case of predictive AI, for example, a credit risk scoring system based on machine learning will make better lending decisions than most humans when presented with simple credit card applications. But if the task is to assess loans involving complex structured finance transactions in which every application is unique, it’s better to let a human decide.
The same holds true for GenAI. A recent study by the BCG Henderson Institute, in collaboration with leading academics , found that GenAI excels at tasks such as creative product innovation and that human efforts to improve or enhance model outputs in these areas often backfired and led to worse results. On the other hand, for tasks falling outside the technology’s current capabilities, such as solving business problems, GenAI underperformed against humans, more often than not hindering the performance of study participants who leveraged the technology.
In other words, GenAI performs best when humans act as complementors of GenAI output, taking over tasks that fall outside AI’s domain of expertise (as in the predictive AI example of credit scoring). But when humans act as enhancers—taking the output and trying to make it better—they can significantly diminish the value of using AI. (See Exhibit 5.)
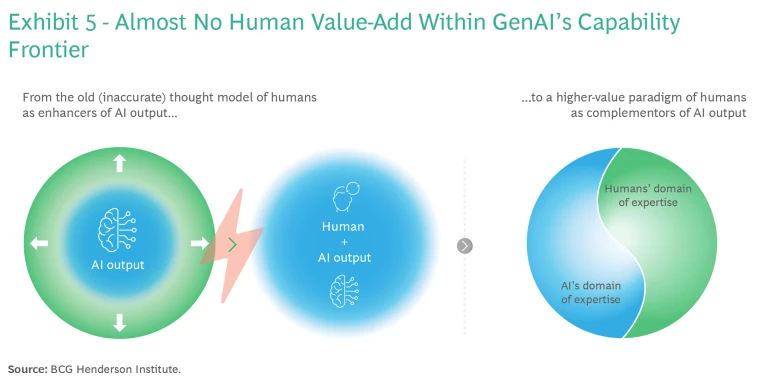
Experimental Discipline
Evaluating and launching smaller-scale use cases within innovation-driven areas of the business can be highly beneficial. Creating these types of AI laboratories can help nurture a broader appetite and greater acceptance for AI solutions within the organization. They also offer a platform to refine new techniques and build technical capabilities. And they provide a practical way to grapple with key decisions, such as whether to develop technological foundations in-house, in-source them, form partnerships, or explore other integration options.
However, the past decade of AI growth and AI experimentation has shown clearly that experimentation can easily get out of hand. A broad “survival of the fittest” approach—that is, launching a large array of small use cases to see which few succeed and flourish—often yields disappointing results. The most effective AI strategies involve conducting selective experiments in controlled laboratory-style testing environments. This approach enables leaders to use insights gained from the experiments to pinpoint a small number of high-impact AI opportunities and rally the organization around them.
As GenAI solutions evolve rapidly, the need for continuous experimentation will remain critical to harnessing their full potential. At the same time, though, a disciplined approach to experimentation is essential.
Reimagine AI-Enabled End-to-End Solutions That Reshape Entire Journeys
The successes and failures of recent AI implementations indicate that companies see greater impact and capture more value when they holistically reimagine entire processes end-to-end and with AI. Isolated use cases that focus on a single part of a larger process can shine brightly for a short time, but they often burn out young, with the scale and impact of change falling short of expectations. And incorporating AI into legacy processes built around the needs and capabilities of human workers can lead to disjointed rollouts and potential friction for employees.
Beyond Tweaking—Transformation
The big wins from AI consistently come from broad transformations that involve rethinking the way an entire process works as part of an AI landscape. An end-to-end approach isn’t a matter of inserting AI at every step, but rather of redesigning processes from the ground up with both AI and human roles in mind for optimized value.
The vast operations of FIs contain a powerful synergy waiting to be unlocked. By leveraging predictive AI and GenAI in concert with human expertise, FIs can achieve enhanced process efficiency and effectiveness—an impact greater than the sum of the parts.
Let’s unpack the roles of the two AI domains within FI workflows:
- Analytical and Predictive Tasks. These left brain tasks, such as determining the best offer with which to reach out to a customer, are appropriate for predictive AI.
- Creative and Expressive Tasks. These right brain tasks, such as creating the content and designing visuals for the customer offer, are better suited for GenAI.
These two simple examples can form the core of a modern hyperpersonalized product marketing campaign. Predictive AI and GenAI work hand in hand to automate most campaign tasks end-to-end, from selecting the target customer to deciding on the many parameters and variables of an offering to writing a tailored message and inserting custom-generated images. But even as AI streamlines many aspects of the workflow, humans remain integral as complementors, supervising the process and dealing with exceptions that require human expertise beyond AI’s capability.
Golden Patterns
Although numerous constellations of AI use are possible, many big opportunities that lie within end-to-end workflows—in particular, opportunities that marry predictive AI and GenAI in complementary ways—follow basic patterns.
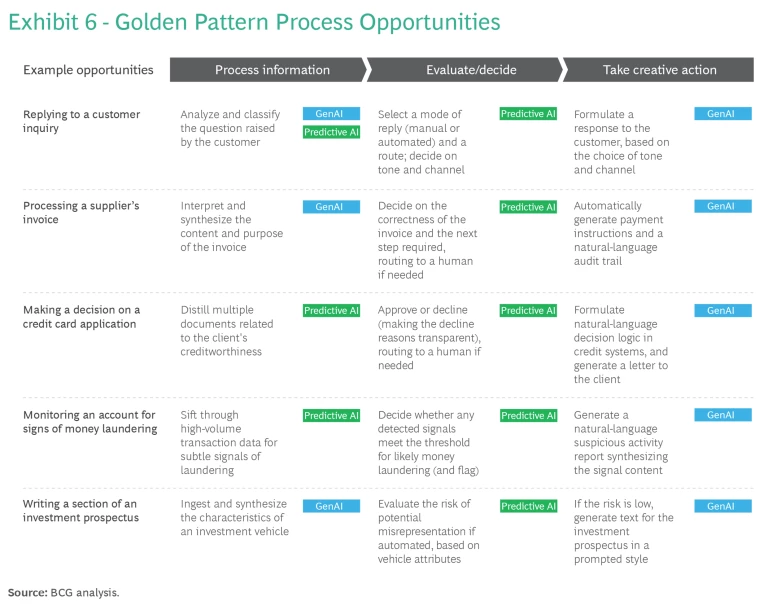
One such pattern consists of three steps: (1) process information; (2) evaluate/decide; (3) take creative action. In practice, this might be the workflow for replying to a customer inquiry, processing a supplier’s invoice, making a decision on a credit card application, monitoring an account for signs of money laundering, or writing a section of an investment prospectus. (See Exhibit 6.)
In legacy processes based on human expertise, a human sifts through the information, evaluates it, comes to a decision, and then takes action. But each of these stages in the pattern is an opportunity for predictive AI and GenAI to team up with the human.
Depending on the specific context, the first step (process information) might offer an opportunity to use GenAI to synthesize and condense large amounts of information into easily digestible summaries, or to engage the power of predictive AI to narrow the field of choices by extracting targeted insights from large data sets.
In the second step (evaluate/decide), a predictive AI model can reliably make automated decisions on cases that lie within its domain of expertise (typically the lion’s share of cases to be decided) and route the exceptional cases to a human in the loop. Here, the predictive model acts as the central steering mechanism for the process, independently determining the need for human involvement.
The third step (take creative action), whether it involves composing a loan rejection letter, a suspicious activity report, or a response to a customer’s question, can often be turned over to a GenAI model—for full automation of simple and/or non-mission-critical cases, or at least for preprocessing of repetitive elements when the occasional imprecision of GenAI is a risk to full automation.
Repetitive, high-volume workflows that follow a golden pattern of this sort in one or more places are game-changing opportunities to transform the process end-to-end.
Focus the Journey on People and Process, Not Just on Tech
Rapid advances in AI make it all too easy to become fixated on the technology, the IT implementation, and the data underlying it. And indeed, leaders face many important challenges here. AI is data-hungry and can lead to uncontrolled data proliferation, so a clear data strategy is essential. And although a GenAI model such as ChatGPT is very user-friendly, it is not at all IT-friendly to implement at scale.
But time and again we see instances where softer success factors—the target operating model and its organizational structures, the approach to AI talent and skills management, and the change management that must accompany any transformation—are underrepresented and underfunded within bank’s AI strategies and prove to be the most critical success factors.
Operating Model and Organizational Structure
AI enables significant productivity growth. Work is automated or augmented, and roles must be redesigned. We see four major types of impact on work that will alter roles across the organization (and drive the many examples listed in Exhibit 4):
- Repetitive tasks such as low-code/no-code automation
- Knowledge synthesis such as review of all commercial loan agreements
- Data-driven decisions such as automation of vendor negotiations
- Creative tasks such as augmentation of code generation
To adjust to this change, FIs must be bold in rethinking people-driven processes and reimagining whole functions. This effort will require the creation of more interdisciplinary teams with embedded data, business analysis, and legal capabilities; the implementation of a flatter and more agile structure for quicker iterations and decisions; and a reduction in spans of control in order to handle the increasingly complex nature of human work.
Finally, a platform operating model is critical to supporting successful AI adoption. An elevated market orientation with greater ability to rapidly deploy people, processes, and data will support faster and more assertive business model innovation and disruption. Cross-functional teams with end-to-end ownership of products, journeys, and services will support reimagining whole processes, and the platform operating model’s ability to drive scalability with standardization and without compromising on customization will be a key enabler.
Talent and Skills
Going forward, nearly every human role will have a relationship with AI:
- Roles that build AI such as technology specialists who create and monitor AI models and support tech platforms, leveraging deep technical capabilities
- Roles that shape AI such as functional experts who direct AI operations to deliver business outcomes and integrate models into business processes
- Roles that use AI such as practitioners who work with outputs from AI models, interpreting resulting content and data to deliver value to customers and employees
- Roles that govern AI such as specialists who monitor AI output to ensure that the software drives returns and to verify that the system uses tech safely and ethically
GenAI will have a high degree of impact on certain functions, including marketing, customer service, legal, and software development. These functions are likely to see extensive automation, resulting in significant opportunities for cost reduction, demand generation via higher-quality service, and the ability to focus resources on higher-value tasks.
Financial institutions must be pragmatic about implementing changes. This entails identifying which roles have the highest value to their particular GenAI strategy and then developing an appropriate value-added talent plan. (See Exhibit 7.) To manage the transition to GenAI well across all functions, executives must integrate GenAI directly into their workforce planning process, defining skills required in the future state, assessing current workforce potential, devising strategies for filling supply-demand gaps, and supporting comprehensive culture and change management to inform the organization’s “build, buy, or borrow” talent strategies.
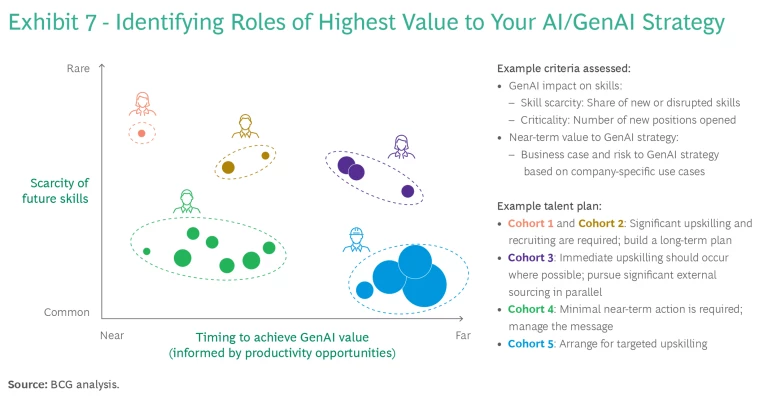
Prioritize Governance, Defining Your Own Rules of the Road
Achieving transformative impact from AI and gaining acceptance of and trust for AI solutions within the organization become possible only when the safeguards of a strong AI governance framework are in place. Without solid governance, both predictive AI and GenAI can easily fall afoul of legal, regulatory, and reputational hazards. The risk of bias against certain customers, for example, may increase with large language models (LLMs) that train on biased public data sets obtained from the internet. Company leaders are struggling with this difficulty, as a recent BCG survey of 2,000 global executives found. Fully 70% of respondents said that concerns about the limited traceability of sources of LLMs discouraged them from using GenAI, and 68% said that fear of the black box nature of the technology and the increased risk of data breaches held back their implementation of GenAI.
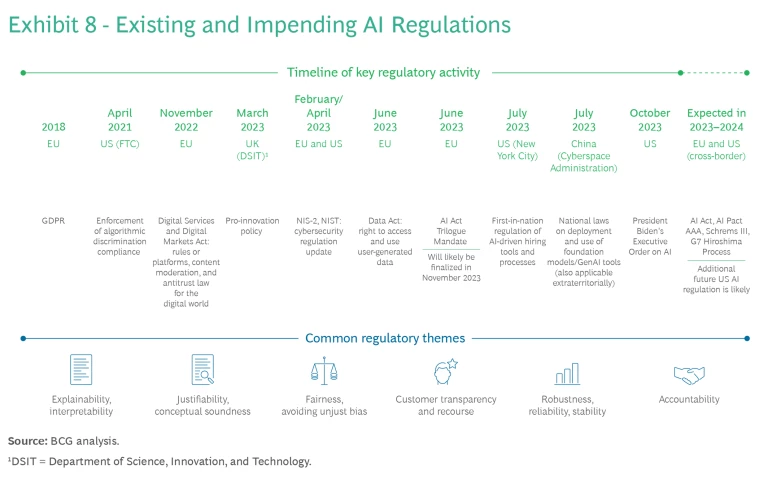
Regulators around the globe have been busy finalizing specific AI laws, amending them with GenAI provisions, and updating data privacy, liability, and copyright laws for the new technology. (See Exhibit 8.) But the technology and its effects are evolving faster than ever, so regulatory uncertainty around GenAI is likely to prevail for some time. Nevertheless, three frameworks are particularly noteworthy for financial institutions. FIs should expect to receive special scrutiny in all three of these regimes, as their products are considered essential to citizens and particularly sensitive.
The first and second regimes are the upcoming ASEAN Guide in AI Governance and Ethics (a guiding framework) and, much more importantly, the EU’s AI Act (a risk-based consumer protection law that is the first horizontal law on AI in the world). The EU AI Act classifies predictive AI and GenAI applications into four risk categories. Applications that fall into the “unacceptable risk” category will be banned from the European market, while applications that fall into the “high-risk” category will be subject to pre- and post-deployment barriers and obligations. Common predictive AI creditworthiness assessments will likely be high-risk applications, as will GenAI-powered customer support chatbots. Still in its final negotiation stages, the EU AI Act is supposed to reach final form by the end of 2023 or early 2024 and, after a grace period, will apply to all products in the European market. Failure to conform to its requirements may result in fines of up to 7% of global annual turnover.
The third regime is the US regulators’ approach, which currently aims to adapt existing regulations rather than to create new laws, and which takes a more national-security-driven perspective on GenAI risks. President Joe Biden’s executive order on AI issued on October 30, 2023, sets in motion a sector-specific set of checks and balances, along with measures to foster the safe and responsible use of the technology by companies and by the government itself. It is the first step toward legislation, but when and how the US will regulate AI remains a subject of debate in Congress and within the administration.
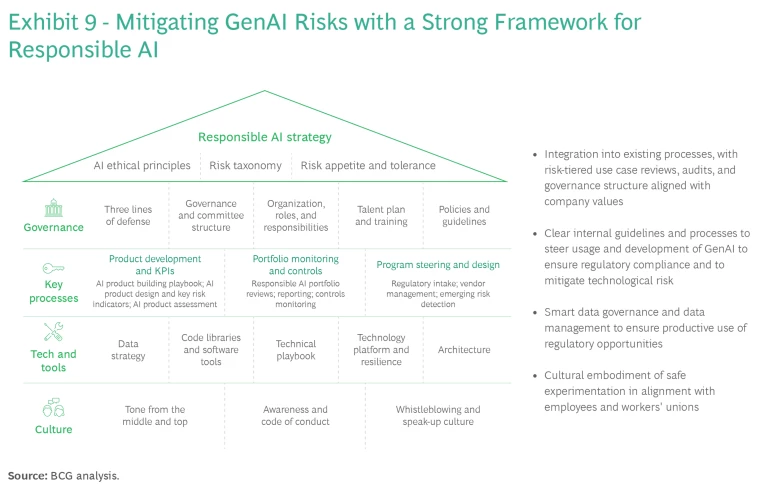
With appropriate guardrails in place to guide AI developers and users, companies should be able to deploy and quickly scale even rapidly changing technologies, with clear controls on the risks and with high regulatory compliance. These guardrails should center on a framework that ensures alignment of AI development and operation with the bank’s purpose and values while still delivering transformative business impact. We call this approach responsible AI. (See Exhibit 9.)
A holistic and agile
responsible AI
framework must include five key components:
- Strategy—a comprehensive AI strategy linked to the firm's values as well as to its risk strategy and ethical principles
- Governance—oversight by a defined responsible AI leadership team, with established escalation paths to identify and mitigate risks
- Processes—rigorous processes put in place to monitor and review products to ensure that they meet responsible AI criteria
- Technology— data and technology infrastructure established to mitigate AI risks, including toolkits to support responsible AI by design and appropriate life-cycle monitoring and management
- Culture—strong understanding among all staff, including AI developers and users, of their roles and duties in upholding responsible AI, and strict adherence to them
A recent BCG study in collaboration with MIT Sloan Management Review found that organizations that successfully integrate responsible AI practices into the full AI product life cycle realize more meaningful benefits. In fact, the likelihood of making full use of the benefits of predictive AI nearly triples, jumping from 14% to 41%, when companies become leaders in responsible AI.
The rise of AI in the workplace will undoubtedly surface complex and pressing questions related to human-AI collaboration and will probably elicit strong positions from workers’ unions on process changes and technology implementation. Questions will arise that the new AI regulations do not answer. But executives who prepare for this eventuality now by developing a holistic RAI framework will have a critical advantage and will set up their AI transformations for success.
Aim for the Horizon
Like any foundational new technology , GenAI raises numerous important issues—around how to realize opportunities for greater efficiency and effectiveness, but also around how to deploy the technology, how to address the complexities of a new people strategy, and how to keep the technology within the bounds of safe regulation and good governance.
The temptation to wait and see may be strong, but too much is at stake to play the short game. Executives must make investigating and adopting AI, including GenAI, a transformational priority for their organizations, taking a medium- to long-term perspective in their AI strategies, their HR planning, and their approach to building a robust governance framework around the technology. Players that actively plan today for the impending AI revolution in their ways of working will be at a decisive advantage going forward.